Image classification method based on multi-scale dense convolutional neural network and spectral attention mechanism
A technology of convolutional neural network and classification method, which is applied to biological neural network models, neural architectures, computer components, etc., can solve the problem of complex space-spectral features of hyperspectral images extracted by deep models, difficulties in hyperspectral image labeling, and dimensionality reduction processing Problems such as large amount of calculation, to alleviate the problem of gradient disappearance, effective feature utilization, and small sample requirements
- Summary
- Abstract
- Description
- Claims
- Application Information
AI Technical Summary
Problems solved by technology
Method used
Image
Examples
Embodiment Construction
[0031] Now in conjunction with embodiment, accompanying drawing, the present invention will be further described:
[0032] The technical solution of the present invention is a small-sample hyperspectral image classification method based on a multi-scale dense convolutional neural network and a spectral attention mechanism. The concrete measures of this technical scheme are as follows:
[0033] Step 1: Data preprocessing. The hyperspectral image data to be processed is subjected to maximum and minimum normalization.
[0034] Step 2: Data Segmentation. Count the number of labeled samples of each category in the hyperspectral image to be processed, and then extract 5 labeled samples from each category in proportion as training data, and the rest as test data.
[0035] Step 3: Build a network model. Construct a deep network model based on multi-scale dense convolutional neural network and spectral attention mechanism.
[0036] Step 4: Train the network model. The training da...
PUM
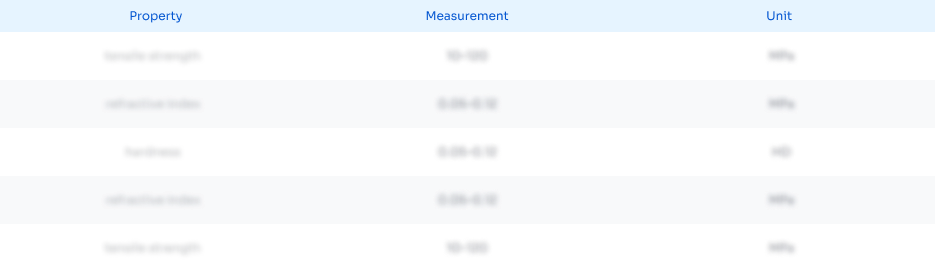
Abstract
Description
Claims
Application Information
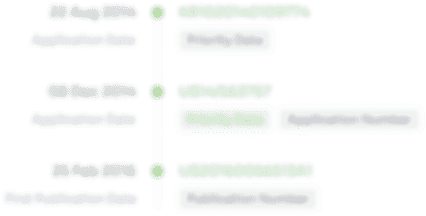
- R&D Engineer
- R&D Manager
- IP Professional
- Industry Leading Data Capabilities
- Powerful AI technology
- Patent DNA Extraction
Browse by: Latest US Patents, China's latest patents, Technical Efficacy Thesaurus, Application Domain, Technology Topic, Popular Technical Reports.
© 2024 PatSnap. All rights reserved.Legal|Privacy policy|Modern Slavery Act Transparency Statement|Sitemap|About US| Contact US: help@patsnap.com