A video saliency target detection method based on a cascade convolutional network and optical flow
A convolutional network and object detection technology, applied in the field of video salient object detection based on cascaded convolutional network and optical flow, can solve the problems of slow speed, high computational complexity, easy loss of edge information, etc., and achieve improved detection speed , clear edges, fine grained effect
- Summary
- Abstract
- Description
- Claims
- Application Information
AI Technical Summary
Problems solved by technology
Method used
Image
Examples
Embodiment Construction
[0034] Now in conjunction with embodiment, accompanying drawing, the present invention will be further described:
[0035] Step 1 Build a cascaded network structure
[0036] The original image is down-sampled to obtain three images of different scales, which are the original image (high scale), the image downsampled by 2 times (medium scale), and the image downsampled by 4 times (low scale). For low-scale images, after 5 convolutional blocks, each convolutional block contains 3 convolutional layers, and the last layer of the first three convolutional blocks each contains a pooling layer with a step size of 2, resulting in a 32-fold downsampling The feature map F1, F1 obtains the saliency map S1 of the low-scale image after 2 times upsampling and the SoftMax layer; the medium-scale image passes through 3 convolutional blocks, and each convolutional block contains 3 convolutional layers and a step size A pooling layer of 2, and then a dilated convolutional layer with a step siz...
PUM
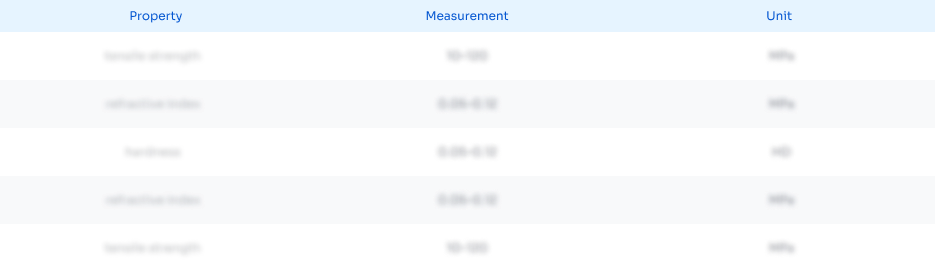
Abstract
Description
Claims
Application Information
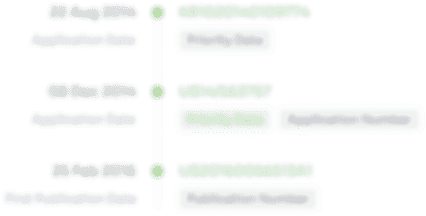
- R&D Engineer
- R&D Manager
- IP Professional
- Industry Leading Data Capabilities
- Powerful AI technology
- Patent DNA Extraction
Browse by: Latest US Patents, China's latest patents, Technical Efficacy Thesaurus, Application Domain, Technology Topic, Popular Technical Reports.
© 2024 PatSnap. All rights reserved.Legal|Privacy policy|Modern Slavery Act Transparency Statement|Sitemap|About US| Contact US: help@patsnap.com