Small target detection method based on feature fusion and depth learning
A technology of small target detection and feature fusion, which is applied in the direction of character and pattern recognition, instruments, computer parts, etc., can solve the problems of high detection accuracy, impossibility, and real-time reduction, so as to improve detection accuracy, improve detection effect, The effect of high detection accuracy
- Summary
- Abstract
- Description
- Claims
- Application Information
AI Technical Summary
Problems solved by technology
Method used
Image
Examples
Embodiment 1
[0023] Target detection is an important research topic in the field of image applications, such as crop detection in smart agriculture, park security detection in the placement field, and so on. At present, there are still many problems in the detection of small targets in images, such as being easily affected by factors such as illumination, rotation, and image scale, but the detection of small targets is very meaningful. For example, using drones for aerial photography, objects in aerial images are often Relatively small, the detection of small targets is an urgent problem to be solved, so the present invention proposes a depth model for small target detection.
[0024] The present invention is a small target detection method based on feature fusion and deep learning, see figure 1 , including the following steps:
[0025] (1) Prepare the atlas: use the training data set of the image set PASCAL VOC2007 and PASCAL VOC2012 as the training set, and use the test data set of the ...
Embodiment 2
[0032] The small target detection method based on feature fusion and deep learning is the same as embodiment 1, and the small target detection network model based on feature fusion and deep learning is set up in step (2) of the present invention, see figure 2 , follow the steps below:
[0033] (2a) Use the first conv4_3 layer of the residual network ResNet101 to build the basic network: increase the connection between non-adjacent but the same resolution layers in the residual network to form the basic network. The input of the basic network is the image to be detected, which is used to extract the image into a feature map of each scale. Since the connection between layers that are not adjacent but have the same resolution is added in the residual network, the loss of information after the convolution operation and the activation function is effectively prevented, and the problems of gradient disappearance and gradient explosion in the deep network model are reduced. , so it...
Embodiment 3
[0038] The small target detection method based on feature fusion and deep learning is the same as in embodiment 1-2, and the construction of a feature pyramid network structure described in step 2c is used to realize multi-scale target detection in target detection, see figure 2 , including:
[0039] (2c1) Build a feature pyramid based on multi-scale feature maps from the conv3, conv5, conv6, conv7, conv8, conv9 network layers in the feature extraction network.
[0040] (2c2) Use the deconvolution operation to upsample the feature maps of the high-level and low-resolution conv5 and conv6 layers in the pyramid structure to obtain the same resolution as the feature maps of the shallow feature maps conv3 and conv5 layers, and add them according to the elements The method performs feature fusion on the high-level feature map and the shallow feature map, and obtains the feature maps of the conv3 and conv5 layers that are more descriptive after fusion.
[0041] The difficulty of s...
PUM
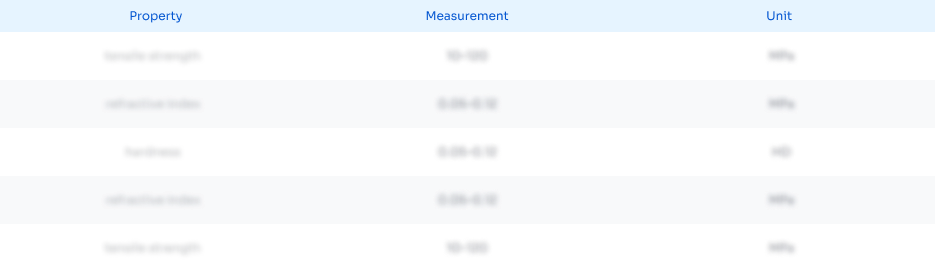
Abstract
Description
Claims
Application Information
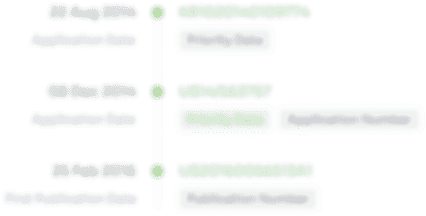
- R&D Engineer
- R&D Manager
- IP Professional
- Industry Leading Data Capabilities
- Powerful AI technology
- Patent DNA Extraction
Browse by: Latest US Patents, China's latest patents, Technical Efficacy Thesaurus, Application Domain, Technology Topic, Popular Technical Reports.
© 2024 PatSnap. All rights reserved.Legal|Privacy policy|Modern Slavery Act Transparency Statement|Sitemap|About US| Contact US: help@patsnap.com