A novel biomedical image automatic segmentation method based on a U-net network structure
A biomedical and network structure technology, applied in the field of automatic segmentation of biomedical images, can solve the problems of unbalanced distribution of positive and negative samples, large contribution of loss function, reduced model generalization ability, etc., so as to improve the segmentation effect and increase the number of pictures. Effect
- Summary
- Abstract
- Description
- Claims
- Application Information
AI Technical Summary
Problems solved by technology
Method used
Image
Examples
Embodiment 1
[0080] In this embodiment, the TensorFlow open source deep learning library is used, NVIDIA Tesla M40GPU is used for acceleration, and the Adam optimization algorithm is used to train the model. The factor is 0.0005) to reduce overfitting; experiments were performed using the Drosophila EM dataset provided by the ISBI 2012 Electron Microscopy Cell Segmentation Challenge.
[0081] The training data set of this embodiment consists of 30 serial slices of central nervous stem cells of the first instar larvae of Drosophila under an electron microscope, each of which contains 512×512 pixels and corresponds to a gold standard for segmentation; Figure 5-6 As shown, in the gold standard image, white represents cells and black represents cell membranes, and the test set consists of another thirty images. Since deep learning requires a large amount of data for training, data enhancement methods such as random flipping, rotation, and elastic distortion are used here to increase the numbe...
Embodiment 2
[0090] In this example, the difference from Example 1 is that the Warwick-QU data set provided by the GLand Segmentation (GLaS) challenge is used for experiments. The data set contains 165 original images (after dyeing), and each image has a corresponding gold standard image marked by experts. Here, 85 images in the training set are used for training, and test set A and test set B are used. authenticating.
[0091] During training, the original image is randomly cut into 512×512 image blocks (when the length or width of the original image is less than 512, it is filled with 0), which not only ensures that the image size in each batch is consistent during training, but also serves as A method of data augmentation to reduce overfitting. In addition, this method uses the same data augmentation method as the Drosophila EM data experiment.
[0092] Here F1 score and Object Hausdorff are used as evaluation indicators, where F1 score is used to evaluate gland detection, and a segme...
PUM
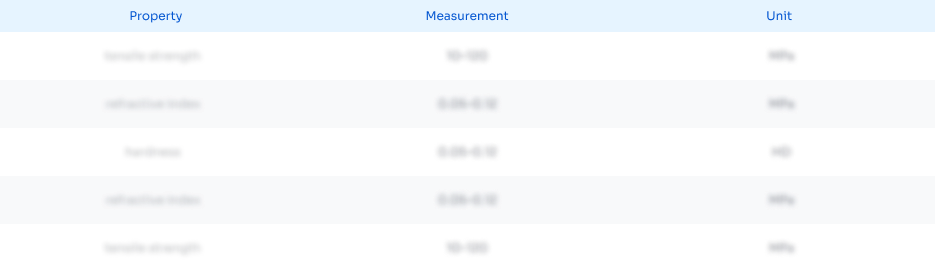
Abstract
Description
Claims
Application Information
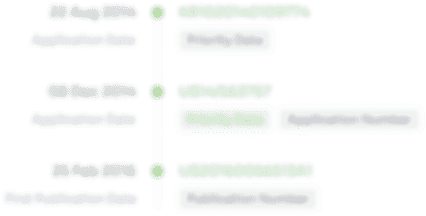
- R&D Engineer
- R&D Manager
- IP Professional
- Industry Leading Data Capabilities
- Powerful AI technology
- Patent DNA Extraction
Browse by: Latest US Patents, China's latest patents, Technical Efficacy Thesaurus, Application Domain, Technology Topic, Popular Technical Reports.
© 2024 PatSnap. All rights reserved.Legal|Privacy policy|Modern Slavery Act Transparency Statement|Sitemap|About US| Contact US: help@patsnap.com