A visual depth estimation method based on depth-differentiable convolutional neural network
A convolutional neural network, convolutional network technology, applied in the field of monocular visual depth estimation, can solve the problems of small share, insufficient feature diversity, loss of object edge information, etc.
- Summary
- Abstract
- Description
- Claims
- Application Information
AI Technical Summary
Problems solved by technology
Method used
Image
Examples
Embodiment Construction
[0033] The present invention will be further described in detail below in conjunction with the accompanying drawings and embodiments.
[0034] A visual depth estimation method based on a deep separable convolutional neural network proposed by the present invention includes two processes of a training phase and a testing phase.
[0035] The specific steps of the described training phase process are:
[0036] Step 1_1: Select N original monocular images and the real depth images corresponding to each original monocular image, and form a training set, and record the nth original monocular image in the training set as {Q n (x,y)}, combine the training set with {Q n (x,y)} corresponds to the real depth image is recorded as Among them, N is a positive integer, N≥1000, such as N=4000, n is a positive integer, 1≤n≤N, 1≤x≤R, 1≤y≤L, R means {Q n (x,y)} and The width of L means {Q n (x,y)} and height, R and L are divisible by 2, Q n (x,y) means {Q n The pixel value of the pixe...
PUM
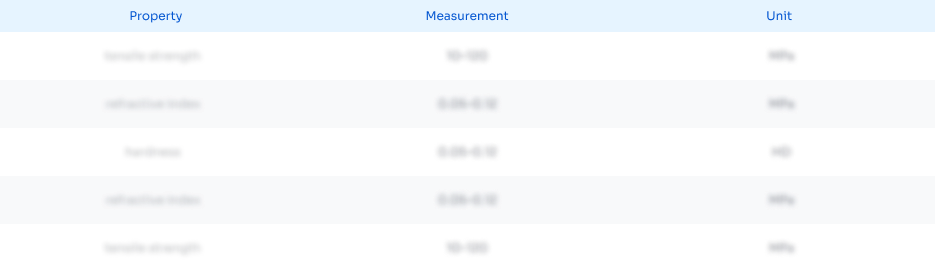
Abstract
Description
Claims
Application Information
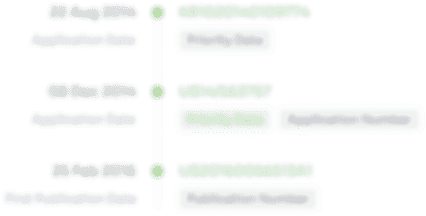
- R&D Engineer
- R&D Manager
- IP Professional
- Industry Leading Data Capabilities
- Powerful AI technology
- Patent DNA Extraction
Browse by: Latest US Patents, China's latest patents, Technical Efficacy Thesaurus, Application Domain, Technology Topic, Popular Technical Reports.
© 2024 PatSnap. All rights reserved.Legal|Privacy policy|Modern Slavery Act Transparency Statement|Sitemap|About US| Contact US: help@patsnap.com