Two-channel convolution neural network semantic segmentation method sensitive to small targets
A convolutional neural network and semantic segmentation technology, applied in the field of dual-channel convolutional neural network semantic segmentation, can solve problems such as unbalanced precision
- Summary
- Abstract
- Description
- Claims
- Application Information
AI Technical Summary
Problems solved by technology
Method used
Image
Examples
Embodiment Construction
[0033] The technical solutions of the present invention will be described in detail below in conjunction with the accompanying drawings.
[0034] Such as figure 1 As shown, a kind of small target sensitive two-channel convolutional neural network semantic segmentation method proposed by the present invention comprises the following steps:
[0035] Step 1, use the Caffe deep learning framework to build a non-weighted learning network and a weighted learning network. Among them, the non-weighted learning network is used to segment the main part of the target in the image, and the weighted learning network is used to segment the small target in the image.
[0036] The algorithm architecture built is as follows figure 2 As shown, the algorithm contains two networks with similar structure: unweighted learning network and weighted learning network. Unweighted learning network means that the network uses ordinary loss function to train the network, which is responsible for segmen...
PUM
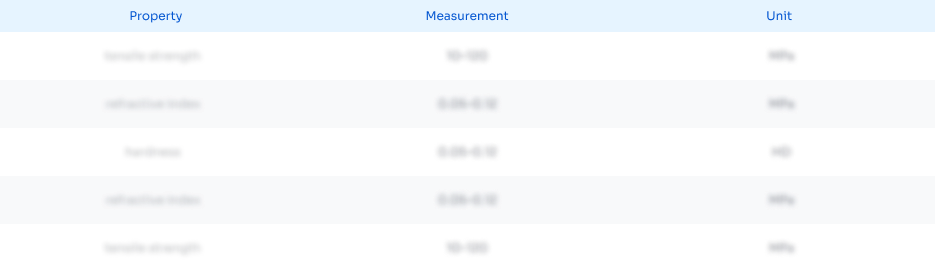
Abstract
Description
Claims
Application Information
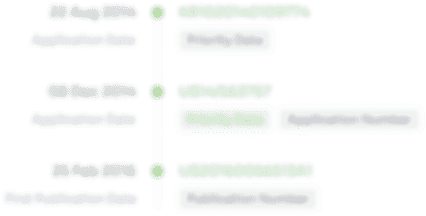
- R&D Engineer
- R&D Manager
- IP Professional
- Industry Leading Data Capabilities
- Powerful AI technology
- Patent DNA Extraction
Browse by: Latest US Patents, China's latest patents, Technical Efficacy Thesaurus, Application Domain, Technology Topic.
© 2024 PatSnap. All rights reserved.Legal|Privacy policy|Modern Slavery Act Transparency Statement|Sitemap