Rail surface defect detection method based on depth learning network
A deep learning network and defect detection technology, applied in the field of deep learning, can solve the problem of less time spent on detection, and achieve the effects of less time-consuming, improved accuracy, and high detection and recognition rate
- Summary
- Abstract
- Description
- Claims
- Application Information
AI Technical Summary
Problems solved by technology
Method used
Image
Examples
Embodiment Construction
[0032] The present invention will be further described below in conjunction with the accompanying drawings and specific embodiments. The present invention is described by taking rail surface defect detection as an example, but the present invention is not limited thereto.
[0033] Such as figure 1 Shown, the method that the present invention proposes comprises the steps:
[0034] Step 1. Manually mark rail defect images and make a data set
[0035] The present invention preprocesses limited rail images. Collected 195 rail sample images, for the accuracy of training, these images are divided into two different types: a total of 128 images of smooth rail surface, a total of 67 images of rough surface of rail. Among these rail pictures, 184 of them were used as the training set for training, including 123 images with smooth rail surfaces and 61 images with rough rail surfaces; 11 images were used as test sets, with 5 images with smooth rail surfaces and 6 images with smooth ra...
PUM
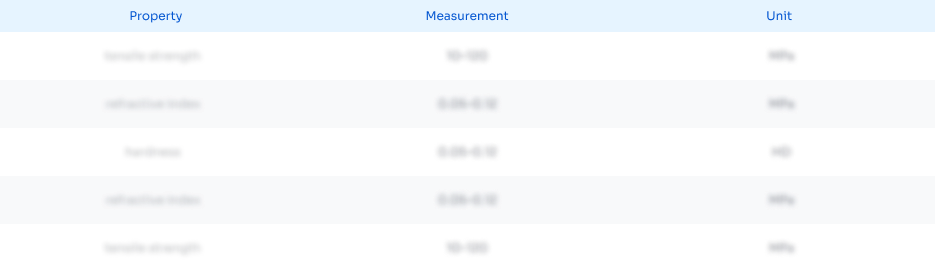
Abstract
Description
Claims
Application Information
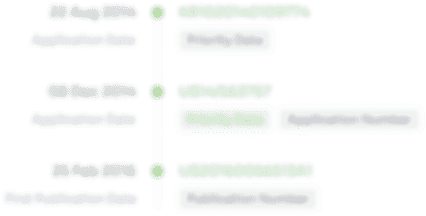
- R&D Engineer
- R&D Manager
- IP Professional
- Industry Leading Data Capabilities
- Powerful AI technology
- Patent DNA Extraction
Browse by: Latest US Patents, China's latest patents, Technical Efficacy Thesaurus, Application Domain, Technology Topic, Popular Technical Reports.
© 2024 PatSnap. All rights reserved.Legal|Privacy policy|Modern Slavery Act Transparency Statement|Sitemap|About US| Contact US: help@patsnap.com