EEG classification method adaptive to different sampling frequencies
A technology of sampling frequency and classification method, applied in applications, medical science, sensors, etc., can solve problems such as difficulty in manual feature design, difficult control of results, and cumbersome data processing.
- Summary
- Abstract
- Description
- Claims
- Application Information
AI Technical Summary
Problems solved by technology
Method used
Image
Examples
Embodiment Construction
[0049] The following embodiments will further illustrate the present invention in conjunction with the accompanying drawings
[0050] Embodiments of the present invention include the following steps:
[0051] 1) Convolutional neural network is a feed-forward neural network that improves the classification ability of patterns through posterior probability. The network mainly includes a convolutional layer, a pooling layer, a fully connected layer and a softmax layer. The convolutional layer performs convolution calculation on the input signal data through different convolution kernels to obtain feature maps (the number of convolution kernels is equal to the number of feature maps. ). The pooling layer is the process of downsampling the feature map obtained by the convolution operation of the previous layer. The network often increases the depth of the network by continuously iterating the convolutional layer and pooling layer, while the fully connected layer fully connects th...
PUM
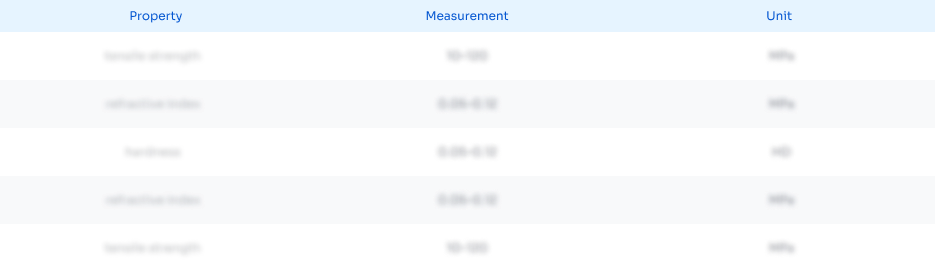
Abstract
Description
Claims
Application Information
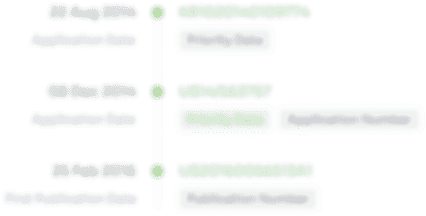
- R&D Engineer
- R&D Manager
- IP Professional
- Industry Leading Data Capabilities
- Powerful AI technology
- Patent DNA Extraction
Browse by: Latest US Patents, China's latest patents, Technical Efficacy Thesaurus, Application Domain, Technology Topic, Popular Technical Reports.
© 2024 PatSnap. All rights reserved.Legal|Privacy policy|Modern Slavery Act Transparency Statement|Sitemap|About US| Contact US: help@patsnap.com