Hyperspectral image classification based on set empirical mode decomposition of image features
A technology that integrates empirical modalities and hyperspectral images. It is applied in character and pattern recognition, pattern recognition in signals, and instruments. The effect of solving extraction difficulties, reducing misclassification, and improving classification accuracy
- Summary
- Abstract
- Description
- Claims
- Application Information
AI Technical Summary
Problems solved by technology
Method used
Image
Examples
Embodiment Construction
[0043] figure 1 It is a schematic diagram of the hyperspectral image classification method based on the set empirical mode decomposition of image features proposed by the present invention. The input is a hyperspectral image and a training sample set, and the output is a classification result map. Such as figure 1 As shown, the specific implementation details of each part of the present invention are as follows:
[0044] S1. Use principal component analysis to remove redundant information in hyperspectral images, and at the same time perform band optimization and data dimensionality reduction.
[0045] Different from grayscale images and color images, the original hyperspectral image I usually contains hundreds of bands. Due to the complexity and redundancy of information in different bands, the first K of high-dimensional hyperspectral data is extracted by principal component analysis. The main components are as follows:
[0046] o K =PCA(I)(1)
[0047] where I represent...
PUM
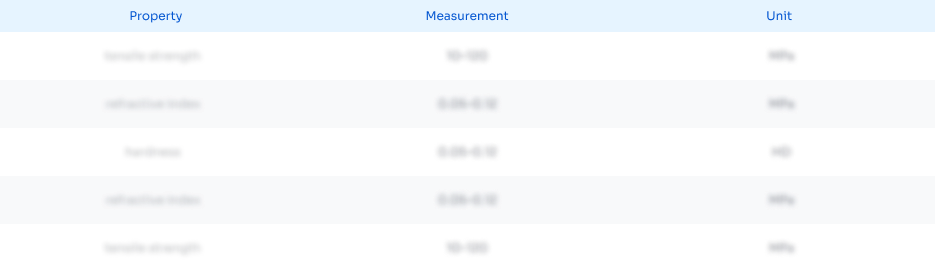
Abstract
Description
Claims
Application Information
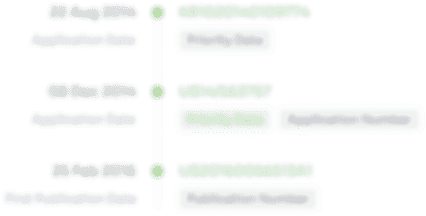
- R&D Engineer
- R&D Manager
- IP Professional
- Industry Leading Data Capabilities
- Powerful AI technology
- Patent DNA Extraction
Browse by: Latest US Patents, China's latest patents, Technical Efficacy Thesaurus, Application Domain, Technology Topic, Popular Technical Reports.
© 2024 PatSnap. All rights reserved.Legal|Privacy policy|Modern Slavery Act Transparency Statement|Sitemap|About US| Contact US: help@patsnap.com