Semantic Generation Method of Remote Sensing Image Based on Fast Region Convolutional Neural Network
A convolutional neural network and remote sensing image technology, applied in the field of image semantic generation, can solve the problems of not being able to get the relationship between the target and the image as a whole, not being able to get the relationship between the target and the target in the image, and the method is not systematic enough.
- Summary
- Abstract
- Description
- Claims
- Application Information
AI Technical Summary
Problems solved by technology
Method used
Image
Examples
Embodiment Construction
[0023] Below in conjunction with accompanying drawing and specific embodiment, the present invention is described in further detail:
[0024] Refer to attached figure 1 , the realization steps of the present invention are as follows.
[0025] Step 1: Construct training sample set and test sample set.
[0026] Download the UCM-Captions Data Set, Sydney-Captions Data Set and RSICD three remote sensing image semantic generation datasets from the website of the State Key Laboratory of Surveying, Mapping and Remote Sensing at Wuhan University, and use 60% of the image-text pairs in each dataset as training samples. The remaining 40% image-text pairs are used as test samples.
[0027] Step 2 uses the fast area convolutional network to extract the image features of the remote sensing images in the training samples:
[0028] The structure of the fast area convolutional network is as follows figure 2 As shown, it contains a region candidate network and a three-layer convolutional ...
PUM
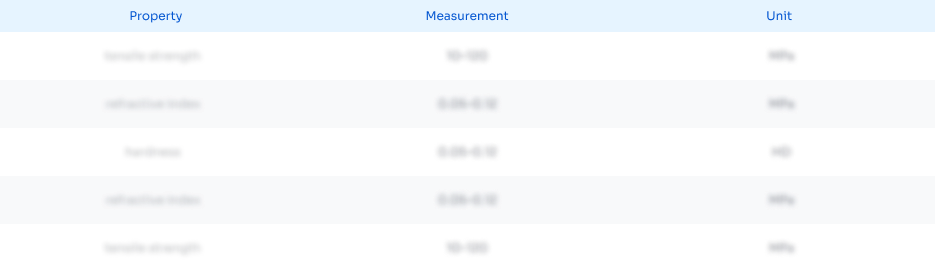
Abstract
Description
Claims
Application Information
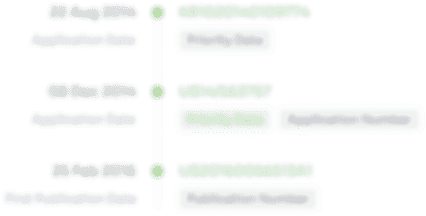
- R&D Engineer
- R&D Manager
- IP Professional
- Industry Leading Data Capabilities
- Powerful AI technology
- Patent DNA Extraction
Browse by: Latest US Patents, China's latest patents, Technical Efficacy Thesaurus, Application Domain, Technology Topic.
© 2024 PatSnap. All rights reserved.Legal|Privacy policy|Modern Slavery Act Transparency Statement|Sitemap