Steel rail tread defect recognition method based on combination of gray image and depth image
A defect recognition, grayscale image technology, applied in image enhancement, image analysis, image data processing and other directions, can solve the problems of large amount of 3D data and slow recognition speed, achieve high-precision defect recognition, low robustness, reduce The effect of false positives
- Summary
- Abstract
- Description
- Claims
- Application Information
AI Technical Summary
Problems solved by technology
Method used
Image
Examples
Embodiment Construction
[0037] The basic idea of the present invention is: using the registered rail tread gray image and depth image, using the convolutional neural network, regression output the type distribution probability of the target defect and the position coordinates in the image, that is, to realize the defect recognition of the rail tread .
[0038] In the following, the present invention will be further described in detail in conjunction with specific embodiments and an end-to-end convolutional neural network that predicts the position and category of the output tread defect from the input registered rail tread gray image and depth image as an example.
[0039] Such as figure 1 As shown, the present invention is based on the rail tread defect recognition method that grayscale image and depth image combine and comprises the following steps:
[0040] Step 11: Build a dataset of rail tread images
[0041] The rail tread image dataset contains the registered rail tread gray image and dept...
PUM
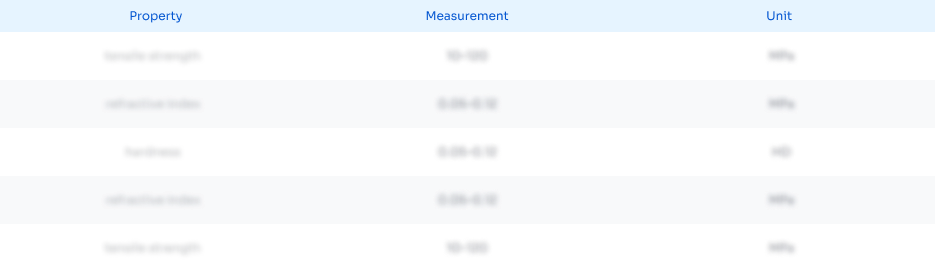
Abstract
Description
Claims
Application Information
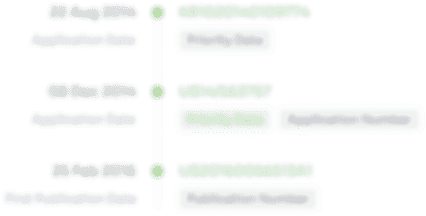
- R&D Engineer
- R&D Manager
- IP Professional
- Industry Leading Data Capabilities
- Powerful AI technology
- Patent DNA Extraction
Browse by: Latest US Patents, China's latest patents, Technical Efficacy Thesaurus, Application Domain, Technology Topic, Popular Technical Reports.
© 2024 PatSnap. All rights reserved.Legal|Privacy policy|Modern Slavery Act Transparency Statement|Sitemap|About US| Contact US: help@patsnap.com