Progressive block knowledge distillation method for neural network acceleration
A technology of neural network and distillation method, which is applied in the field of deep network model compression and acceleration, can solve problems such as instability, difficulty in non-joint optimization process, and inability to protect the receptive field information of atomic network blocks well, so as to achieve simple implementation and reduce difficulty Effect
- Summary
- Abstract
- Description
- Claims
- Application Information
AI Technical Summary
Problems solved by technology
Method used
Image
Examples
Embodiment
[0063] The following simulation experiments are carried out based on the above method. The implementation method of this embodiment is as described above, and the specific steps are not described in detail. The following only demonstrates its effects based on the experimental results.
[0064] This embodiment uses the original complex VGG-16 network used for image classification tasks on the CIFAR100 and ImageNet data sets. First, the VGG-16 is divided into 5 teacher sub-network blocks, and then compression and acceleration based on the method of the present invention are carried out.
[0065] The implementation effects are shown in Table 1 and Table 2. As shown in Table 1, on the CIFAR100 data set, the present invention compresses the original model (OriginalVGG). When the parameters of the original model are reduced by 40% and the amount of calculation is reduced by 169%, the top-1 accuracy of the model is It only dropped by 2.22% and the Top-5 accuracy rate dropped by only 1.89%...
PUM
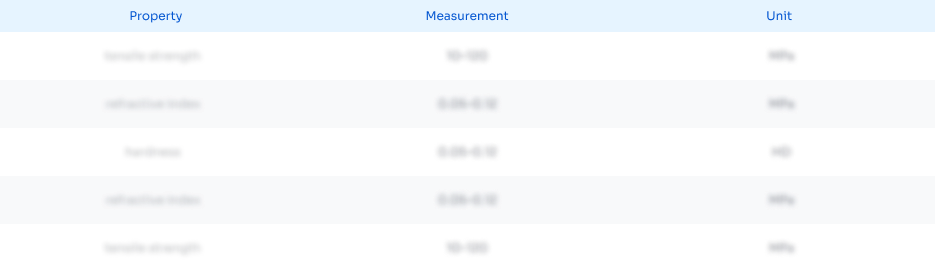
Abstract
Description
Claims
Application Information
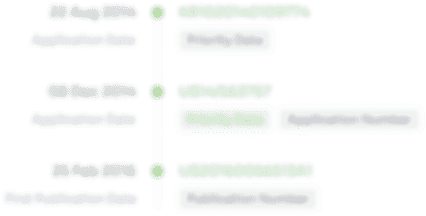
- R&D Engineer
- R&D Manager
- IP Professional
- Industry Leading Data Capabilities
- Powerful AI technology
- Patent DNA Extraction
Browse by: Latest US Patents, China's latest patents, Technical Efficacy Thesaurus, Application Domain, Technology Topic, Popular Technical Reports.
© 2024 PatSnap. All rights reserved.Legal|Privacy policy|Modern Slavery Act Transparency Statement|Sitemap|About US| Contact US: help@patsnap.com