An end-to-end impact crater detection and recognition method based on a fully convolutional neural network structure
A convolutional neural network structure technology, applied in the field of end-to-end impact crater detection and recognition based on a fully convolutional neural network structure, can solve the impact crater target position detection error and apparent diameter detection error that are extremely sensitive and take up storage space Large, time-consuming search and matching, etc., to achieve the effect of reaching the leading performance level, occupying a small space, and enhancing the robustness of recognition
- Summary
- Abstract
- Description
- Claims
- Application Information
AI Technical Summary
Problems solved by technology
Method used
Image
Examples
Embodiment Construction
[0043] The present invention will be further described below in conjunction with the accompanying drawings and specific embodiments.
[0044] 1) Network structure
[0045] The network system proposed by the present invention, called CraterIDNet, is an end-to-end fully convolutional neural network model. The whole system is an independent and unified impact crater detection and identification network. Network structure such as figure 1 Shown:
[0046] CraterIDNet accepts input remote sensing images of any resolution, and outputs the position and diameter of the detected impact craters and the number of the identified impact craters. The network consists of two main parts, the crater detection channel and the crater identification channel. The entire system adopts a fully convolutional architecture without a fully connected layer, which greatly reduces the network scale. In order to further reduce the network scale while ensuring the detection and recognition effect, the p...
PUM
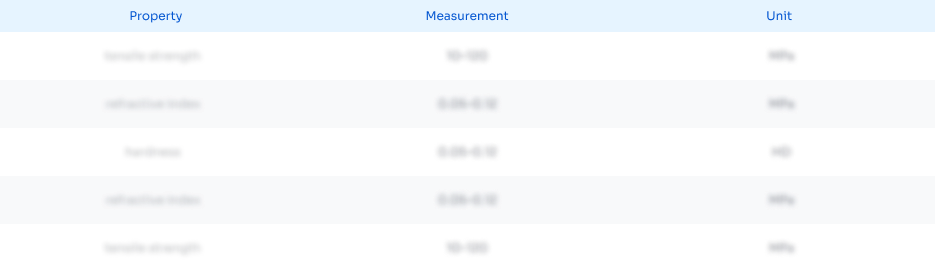
Abstract
Description
Claims
Application Information
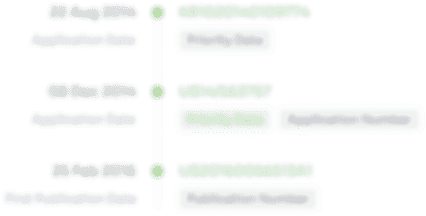
- R&D
- Intellectual Property
- Life Sciences
- Materials
- Tech Scout
- Unparalleled Data Quality
- Higher Quality Content
- 60% Fewer Hallucinations
Browse by: Latest US Patents, China's latest patents, Technical Efficacy Thesaurus, Application Domain, Technology Topic, Popular Technical Reports.
© 2025 PatSnap. All rights reserved.Legal|Privacy policy|Modern Slavery Act Transparency Statement|Sitemap|About US| Contact US: help@patsnap.com