A Deep Value Function Learning Method for Agents Based on State Distribution Perceptual Sampling
A value function and state distribution technology, applied in the field of reinforcement learning, can solve problems such as large differences in quantity, and achieve the effect of improving learning speed, good application value, and improving sample use efficiency
- Summary
- Abstract
- Description
- Claims
- Application Information
AI Technical Summary
Problems solved by technology
Method used
Image
Examples
Embodiment
[0059] The implementation method of this embodiment is as described above, and the specific steps will not be described in detail, and the effect is only shown for case data below.
[0060] First, the hash method is used to reduce the dimensionality and classify the abstract expression of the state set observed by the agent obtained through the convolutional neural network, so as to perceive the distribution of the state space. On this basis, the samples in the empirical data set are reasonably selected. Finally, use the selected sample data to train the value function of the agent, so that it can judge the environment more accurately. The result is as figure 1 , 2 , 3 shown.
[0061] figure 1 After performing the steps S1 and S2 of the present invention for the original empirical data of the present invention, the result of visualizing the samples is a schematic diagram of the distribution of the samples in the state space;
[0062] figure 2 In order to adopt three sam...
PUM
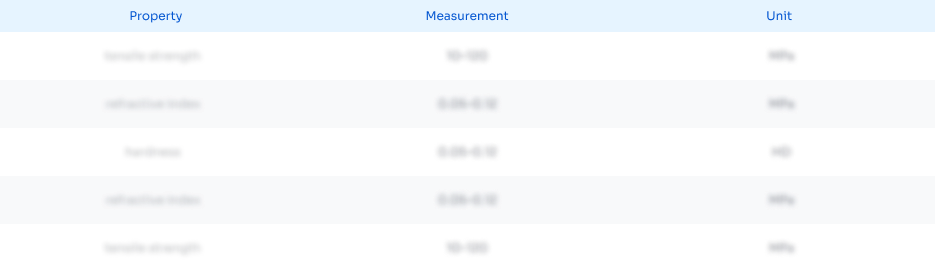
Abstract
Description
Claims
Application Information
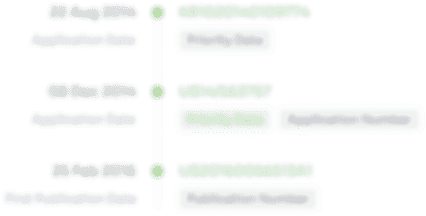
- R&D Engineer
- R&D Manager
- IP Professional
- Industry Leading Data Capabilities
- Powerful AI technology
- Patent DNA Extraction
Browse by: Latest US Patents, China's latest patents, Technical Efficacy Thesaurus, Application Domain, Technology Topic, Popular Technical Reports.
© 2024 PatSnap. All rights reserved.Legal|Privacy policy|Modern Slavery Act Transparency Statement|Sitemap|About US| Contact US: help@patsnap.com