Image denoising method based on mixed robust weights and method noise (MN)
A method of noise and image technology, applied in the field of intelligent information processing, can solve the problem that the NLM algorithm cannot effectively balance the relationship between noise suppression and detail preservation, and achieve the effect of improving accuracy
- Summary
- Abstract
- Description
- Claims
- Application Information
AI Technical Summary
Problems solved by technology
Method used
Image
Examples
Embodiment 1
[0063] Embodiment 1: An image denoising method based on mixed robust weights and method noise, refer to figure 1 ;
[0064] Step 1. Input a noisy image Y={y(i)|i=1,2,...,n}, where y(i) is the gray value of pixel i, and n is the total number of pixels. The noise-containing image Y is pre-denoised by bilateral filtering (BF) algorithm, and the pre-denoising image D is obtained. pre ={d pre (i)|i=1,2,...,n}:
[0065]
[0066]
[0067] Where i, j are the i-th pixel and j-th pixel in the image respectively, N i Represents a neighborhood block centered on pixel i (the size is 5×5), dist(i, j) is the Euclidean distance between pixel i and j, and the parameter σ S and σ G Represent the spatial proximity coefficient and the gray similarity coefficient (the values are respectively taken as 3.0 and 0.7σ, σ is the noise standard deviation of the image), and ω(i) is the normalization item;
[0068] Step 2. Use the non-local mean denoising (HRW-NLM) method based on hybrid robu...
Embodiment 2
[0081] Embodiment 2: effect simulation experiment of the present invention
[0082] Four standard images Lena (512×512), Barbara (512×512), Peppers (256×256), House (256×256) are used for testing, and the mean value is 0 and the standard deviation σ is 15 and 25 respectively. , 35, 50, 80, 100 Gaussian white noise. The simulation experiment is mainly carried out from the following two aspects: the performance comparison of the three NLM algorithms (NLM, HRW-NLM and the inventive method HRWIMN-TSNLM) proposed in the present invention and the comparison between the inventive method (HRWIMN-TSNLM) and other several algorithms ( NLM, MN-TSNLM, BFNLM, MNLM) comparison. The experimental testing environment is MATLAB R2014a.
[0083] The parameters in the simulation experiment are set as follows: the search window size is 7×7, the similarity window size is 3×3, the filter parameter h in NLM=1.0σ, the filter parameter h in HRW-NLM=4.0σ, h in HRWIMN-TSNLM (1) =4.0σ, h(2) =0.5σ(h (1...
PUM
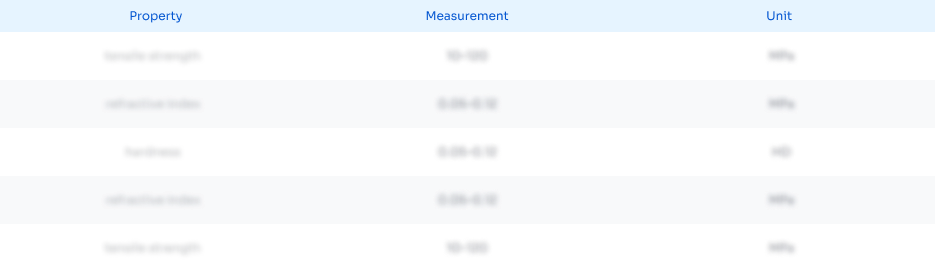
Abstract
Description
Claims
Application Information
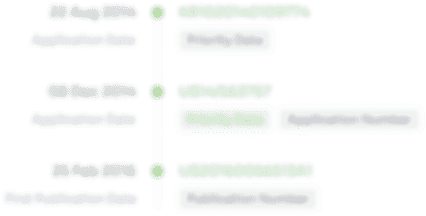
- R&D Engineer
- R&D Manager
- IP Professional
- Industry Leading Data Capabilities
- Powerful AI technology
- Patent DNA Extraction
Browse by: Latest US Patents, China's latest patents, Technical Efficacy Thesaurus, Application Domain, Technology Topic.
© 2024 PatSnap. All rights reserved.Legal|Privacy policy|Modern Slavery Act Transparency Statement|Sitemap