Wind turbine generator bearing fault diagnosing method based on deep joint adaptation network
A technology for fault diagnosis of wind turbines, applied in the field of wind power, can solve problems such as failure to use new fault diagnosis of bearings, and achieve the effect of reducing computational complexity
- Summary
- Abstract
- Description
- Claims
- Application Information
AI Technical Summary
Problems solved by technology
Method used
Image
Examples
Embodiment Construction
[0054] The present invention will be further described below in conjunction with the drawings and embodiments.
[0055] Such as figure 1 As shown, a wind turbine bearing fault diagnosis method based on a deep joint adaptation network includes the following steps:
[0056] 1) Establish a diversified fusion database: Collect wind power system rolling bearing end acceleration data under different working conditions, and at the same time collect wind power system rolling bearing end acceleration data in real time. According to the characteristics of multi-source, heterogeneous, and large noise in the industrial data, all data are collected. After normalization, Fourier transform is used to remove the noise contained in the data, and the processed data under different working conditions are labeled and marked as Source Domain (SD). The labels include normal and inner circle faults. , Outer ring failure, and sphere failure. The real-time collected data after preprocessing is recorded as...
PUM
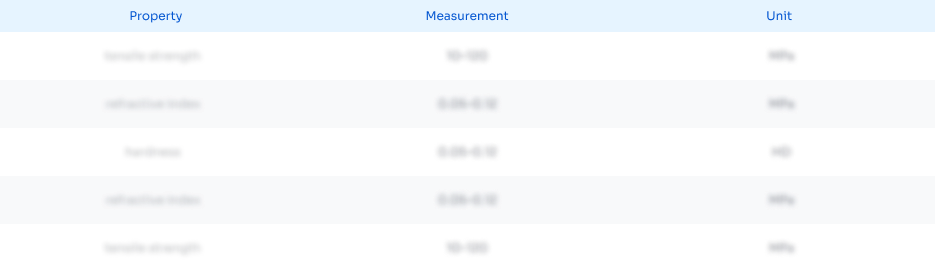
Abstract
Description
Claims
Application Information
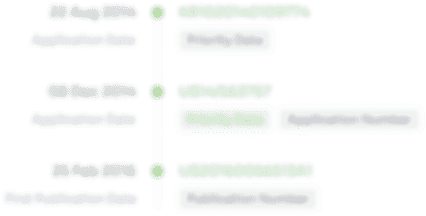
- R&D
- Intellectual Property
- Life Sciences
- Materials
- Tech Scout
- Unparalleled Data Quality
- Higher Quality Content
- 60% Fewer Hallucinations
Browse by: Latest US Patents, China's latest patents, Technical Efficacy Thesaurus, Application Domain, Technology Topic, Popular Technical Reports.
© 2025 PatSnap. All rights reserved.Legal|Privacy policy|Modern Slavery Act Transparency Statement|Sitemap|About US| Contact US: help@patsnap.com