Semantic image segmentation method based on conditional random field graph structure learning
A conditional random field, image segmentation technology, applied in the field of image understanding in computer vision, can solve the problem of poor segmentation effect and achieve good segmentation effect
- Summary
- Abstract
- Description
- Claims
- Application Information
AI Technical Summary
Problems solved by technology
Method used
Image
Examples
Embodiment Construction
[0045] The present invention will be further described below.
[0046] A method for semantic image segmentation based on conditional random field graph structure learning, said method comprising the following steps:
[0047] 1) Train a fully convolutional neural network or use an off-the-shelf fully convolutional neural network for rough segmentation of semantic images;
[0048] 2) Use the rcf neural network to learn the conditional random field graph structure, the process is as follows:
[0049] At present, some people have used richer convolutional features (RCF) to detect object boundaries in images. This embodiment uses the same deep neural network to learn CRF graphs by fine-tuning network parameters on CRF graph data. We first describe the structure of the network, which is actually a modification of the VGG16 network. Modifications include: 1) Cut all fully connected layers and pool5 layers; 2) Each conv layer in VGG16 is connected to a conv layer with core size 1x1...
PUM
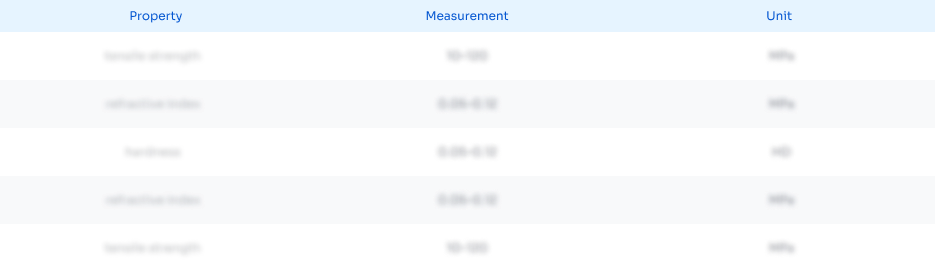
Abstract
Description
Claims
Application Information
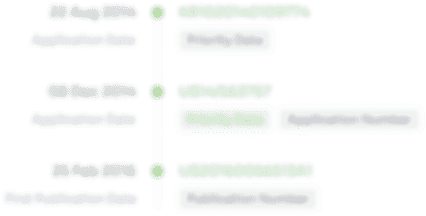
- R&D Engineer
- R&D Manager
- IP Professional
- Industry Leading Data Capabilities
- Powerful AI technology
- Patent DNA Extraction
Browse by: Latest US Patents, China's latest patents, Technical Efficacy Thesaurus, Application Domain, Technology Topic, Popular Technical Reports.
© 2024 PatSnap. All rights reserved.Legal|Privacy policy|Modern Slavery Act Transparency Statement|Sitemap|About US| Contact US: help@patsnap.com