Multi-modal deep leaning classification method based on semi supervision
A technology of deep learning and classification methods, applied in neural learning methods, character and pattern recognition, biological neural network models, etc., can solve problems such as lack of labeled samples
- Summary
- Abstract
- Description
- Claims
- Application Information
AI Technical Summary
Problems solved by technology
Method used
Image
Examples
Embodiment Construction
[0028] Specific embodiments of the present invention will be described in detail below with reference to the accompanying drawings.
[0029] figure 1 The system structure of the embodiment of the present invention is shown. 102 is the input of hyperspectral image samples and label information, 103 represents our preprocessing of received samples, 104 represents our grouping of processed samples, and 105 is sending the grouped samples to semi-supervised multiple Learning in the modal deep learning framework, 106 represents combining the results of each deep learning framework to make a decision process, and 107 represents obtaining the final classification result.
[0030] figure 2 and image 3 The specific practical steps of the semi-supervised multi-modal deep learning framework of the embodiment of the present invention are shown. In this scheme, different modal data of hyperspectral images are fed into the deep neural network, and a semi-supervised method is used to ut...
PUM
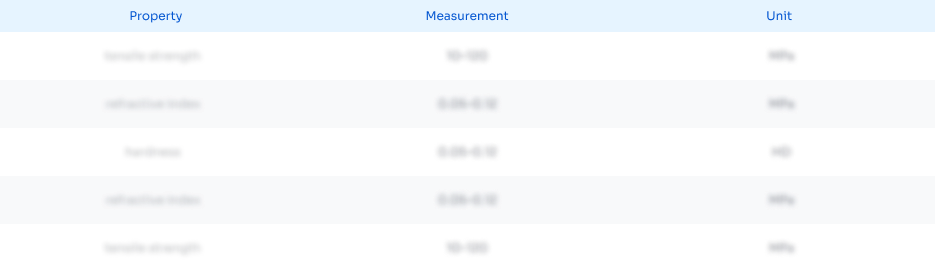
Abstract
Description
Claims
Application Information
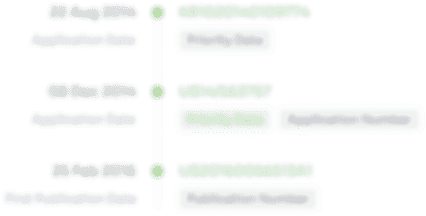
- R&D Engineer
- R&D Manager
- IP Professional
- Industry Leading Data Capabilities
- Powerful AI technology
- Patent DNA Extraction
Browse by: Latest US Patents, China's latest patents, Technical Efficacy Thesaurus, Application Domain, Technology Topic, Popular Technical Reports.
© 2024 PatSnap. All rights reserved.Legal|Privacy policy|Modern Slavery Act Transparency Statement|Sitemap|About US| Contact US: help@patsnap.com