Air valve fault diagnosis method based on HHT and neural network
A fault diagnosis and neural network technology, applied in biological neural network models, neural architectures, testing of mechanical components, etc., can solve the problem of inability to analyze video characteristics such as instantaneous amplitude and instantaneous frequency, and difficulty in obtaining time-frequency information and frequency amplitude. information, it is difficult to reflect the time-varying and non-stationary characteristics of the signal, etc.
- Summary
- Abstract
- Description
- Claims
- Application Information
AI Technical Summary
Problems solved by technology
Method used
Image
Examples
Embodiment Construction
[0017] In order to make the object, technical solution and advantages of the present invention more clear, the present invention will be further described below in conjunction with the accompanying drawings and specific embodiments.
[0018] A kind of compressor valve fault diagnosis method based on HHT feature extraction and RBF neural network provided by the present invention, the flow chart of this method is as follows figure 1 shown, including the following steps:
[0019] Step 1: Use the truncated matrix singular value decomposition method to perform noise reduction preprocessing on the collected vibration signals of the compressor valve under normal and fault conditions. The noise reduction results of one cycle (0.12s) in four working states of the air valve are as follows: figure 2 shown. The specific process is as follows:
[0020] Step 1.1: Select the vibration data of the reciprocating compressor, including normal working state data and three common fault state d...
PUM
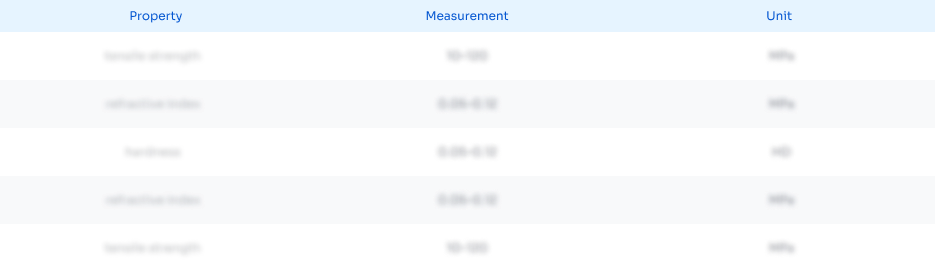
Abstract
Description
Claims
Application Information
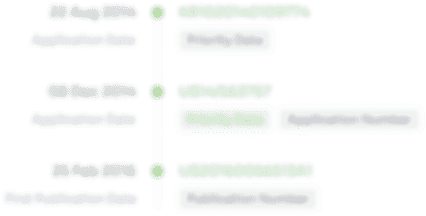
- R&D Engineer
- R&D Manager
- IP Professional
- Industry Leading Data Capabilities
- Powerful AI technology
- Patent DNA Extraction
Browse by: Latest US Patents, China's latest patents, Technical Efficacy Thesaurus, Application Domain, Technology Topic, Popular Technical Reports.
© 2024 PatSnap. All rights reserved.Legal|Privacy policy|Modern Slavery Act Transparency Statement|Sitemap|About US| Contact US: help@patsnap.com