Likelihood-based causal structure learning method
A learning method and likelihood technology, applied in the field of likelihood-based causal structure learning, which can solve problems such as multiple time, cost, and multiple independence tests
- Summary
- Abstract
- Description
- Claims
- Application Information
AI Technical Summary
Problems solved by technology
Method used
Image
Examples
Embodiment Construction
[0041] The specific embodiment of the present invention is described further below:
[0042] A causal structure learning method based on likelihood, comprising the following steps:
[0043] S1), preset causal network structure graph G=(X, D), where X=(x 1 ,x 2 ,...,x n ), x i Indicates the i-th node, D={x i →x j} means x i with x j The directed edge of , if x j for x i The parent node of , then denoted as x i →x j ;
[0044] S2), and define the observation data set O=(o 1 ,o 2 ,...,o n ), where o i =(a 1i ,a 2i ,...a mi ) means the i-th node x i The observation data set of a j,i represents the i-th node x i The jth observation data of ;
[0045] S3), initialize the structure diagram D, and use the observation data of the observation data set O to calculate the initial score S of the structure diagram after the initialization process 0 ;
[0046] S4) Then traverse all the nodes in the initialized structure diagram two by two, and for any two nodes x i w...
PUM
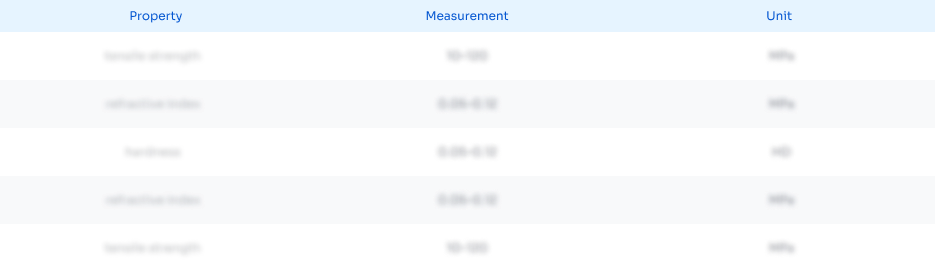
Abstract
Description
Claims
Application Information
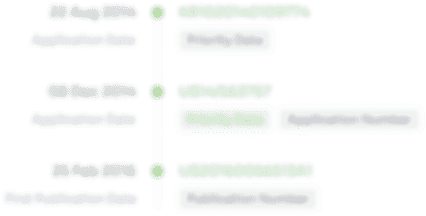
- R&D Engineer
- R&D Manager
- IP Professional
- Industry Leading Data Capabilities
- Powerful AI technology
- Patent DNA Extraction
Browse by: Latest US Patents, China's latest patents, Technical Efficacy Thesaurus, Application Domain, Technology Topic, Popular Technical Reports.
© 2024 PatSnap. All rights reserved.Legal|Privacy policy|Modern Slavery Act Transparency Statement|Sitemap|About US| Contact US: help@patsnap.com