A mixed corpus named entity recognition method based on bi-lstm-cnn
A named entity recognition and corpus technology, applied in the information field, can solve the problems of gradient disappearance, low recognition rate of unregistered words, and insignificant advantages of final named entity recognition results, and achieve the effect of improving accuracy and avoiding unregistered words.
- Summary
- Abstract
- Description
- Claims
- Application Information
AI Technical Summary
Problems solved by technology
Method used
Image
Examples
Embodiment Construction
[0045] In order to make the above objects, features and advantages of the present invention more comprehensible, the present invention will be further described in detail below through specific embodiments and accompanying drawings.
[0046]The invention discloses a Bi-LSTM-CNN-based named entity recognition method of mixed corpus. For example, identifying named entities such as person names, place names, and organization names in corpus data that is mixed in multiple languages. The core problems of the present invention include three: 1. the efficiency of mixed corpus recognition, 2. the precision of named entity recognition, and 3. the recognition precision of unregistered words.
[0047] In order to solve the problem of unregistered words, the present invention abandons the traditional vocabulary method, but adopts the idea based on word vectors, and is based on character vectors rather than word-based vectors.
[0048] In order to solve the problem of low precision of the...
PUM
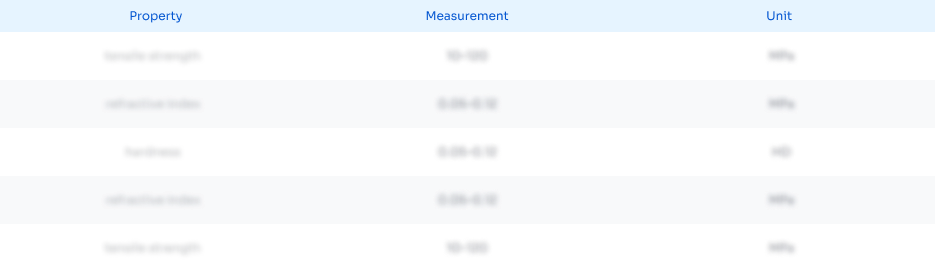
Abstract
Description
Claims
Application Information
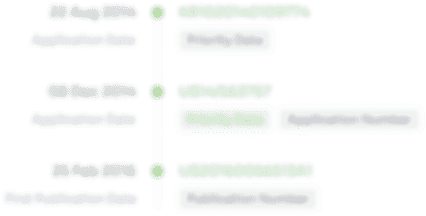
- R&D Engineer
- R&D Manager
- IP Professional
- Industry Leading Data Capabilities
- Powerful AI technology
- Patent DNA Extraction
Browse by: Latest US Patents, China's latest patents, Technical Efficacy Thesaurus, Application Domain, Technology Topic, Popular Technical Reports.
© 2024 PatSnap. All rights reserved.Legal|Privacy policy|Modern Slavery Act Transparency Statement|Sitemap|About US| Contact US: help@patsnap.com