Alzheimer's disease classification method based on depth neural network and multi-mode images
A technology of deep neural network and classification method, applied in the field of Alzheimer's disease classification based on deep neural network and multimodal imaging, can solve the problems of low classification accuracy, incomplete feature extraction, and difficulty in feature extraction of machine learning classification methods. , to avoid loss and facilitate early diagnosis
- Summary
- Abstract
- Description
- Claims
- Application Information
AI Technical Summary
Problems solved by technology
Method used
Image
Examples
Embodiment Construction
[0037] All features disclosed in this specification, or steps in all methods or processes disclosed, may be combined in any manner, except for mutually exclusive features and / or steps.
[0038] Combine below Figure 1-4 The present invention will be described in detail.
[0039] A classification method for Alzheimer's disease based on a deep neural network and multimodal images, comprising the following steps:
[0040] Step 1: Preprocessing N types of images of known categories to obtain N types of 2D image slices and N types of 3D image blocks, the images include training samples and test samples.
[0041]Specifically: collecting enough multimodal information of AD, MCI and NC groups, the multimodal information includes image and non-image features, and the image categories include: MRI images, PET images and DTI images. Perform preprocessing operations such as segmentation, registration, and standardization on the images to obtain MRI gray matter images, PET whole-brain im...
PUM
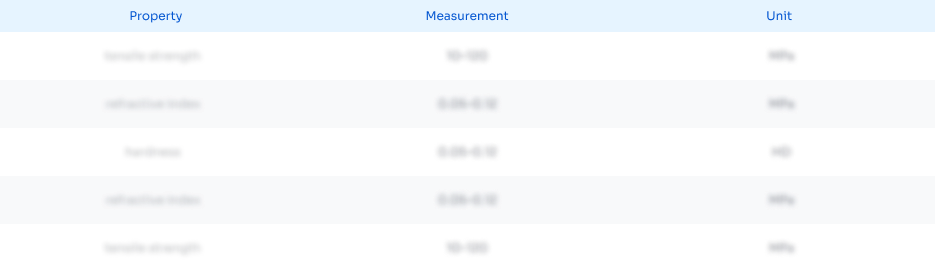
Abstract
Description
Claims
Application Information
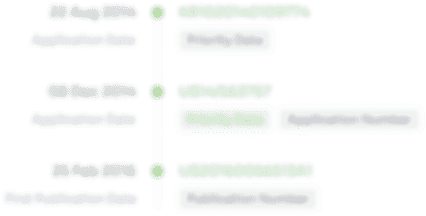
- R&D
- Intellectual Property
- Life Sciences
- Materials
- Tech Scout
- Unparalleled Data Quality
- Higher Quality Content
- 60% Fewer Hallucinations
Browse by: Latest US Patents, China's latest patents, Technical Efficacy Thesaurus, Application Domain, Technology Topic, Popular Technical Reports.
© 2025 PatSnap. All rights reserved.Legal|Privacy policy|Modern Slavery Act Transparency Statement|Sitemap|About US| Contact US: help@patsnap.com