A method for human behavior recognition based on 3D deep convolutional network
A technology of deep convolution and convolutional neural network, applied in the field of computer vision video recognition, can solve problems such as incapable of spatial scale and duration video processing, lack of behavior information, etc., to increase the scale of video training data, improve robustness, improve The effect of completeness
- Summary
- Abstract
- Description
- Claims
- Application Information
AI Technical Summary
Problems solved by technology
Method used
Image
Examples
Embodiment Construction
[0036] In order to make the object, technical solution and advantages of the present invention more clear, the present invention will be further described in detail below in conjunction with the examples. It should be understood that the specific embodiments described here are only used to explain the present invention, not to limit the present invention.
[0037] For action recognition in video, the traditional method turns this problem into a multi-classification problem, and proposes different video feature extraction methods. However, traditional methods extract based on low-level information, such as from visual texture information or motion estimation in videos. Since the extracted information is single, it cannot represent the video content well, and the optimized classifier is not optimal. As a technology in deep learning, convolutional neural network integrates feature learning and classifier learning as a whole, and is successfully applied to behavior recognition in...
PUM
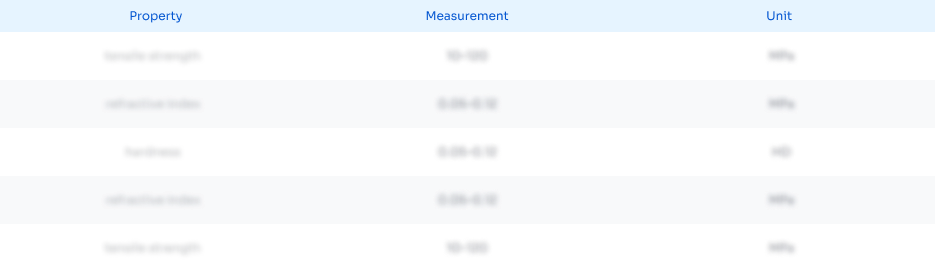
Abstract
Description
Claims
Application Information
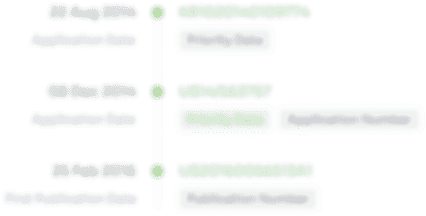
- R&D Engineer
- R&D Manager
- IP Professional
- Industry Leading Data Capabilities
- Powerful AI technology
- Patent DNA Extraction
Browse by: Latest US Patents, China's latest patents, Technical Efficacy Thesaurus, Application Domain, Technology Topic.
© 2024 PatSnap. All rights reserved.Legal|Privacy policy|Modern Slavery Act Transparency Statement|Sitemap