Classification model construction method and device used for macula degeneration region segmentation
A macular degeneration and region segmentation technology, applied in the field of medical image processing, can solve the problems of less application research on fundus image segmentation, lack of strong discrimination and description ability, and inability to obtain segmentation results.
- Summary
- Abstract
- Description
- Claims
- Application Information
AI Technical Summary
Problems solved by technology
Method used
Image
Examples
Embodiment 1
[0075] A classification model construction method for macular lesion region segmentation in fundus images, such as figure 1 shown, including the following steps:
[0076] Step 1: selecting multiple fundus images, performing grayscale processing on them to obtain multiple grayscale images, and sampling the foreground and background of the grayscale images respectively to obtain samples;
[0077] Step 2: Obtain the transformation matrix by using the generalized low-rank approximation method, and perform dimensionality reduction processing on the sample based on the transformation matrix to obtain the low-rank approximate matrix of the sample;
[0078] Step 3: adding label information to the low-rank approximate matrix of the sample as supervision, and constructing a regularization term based on the low-rank approximate matrix and label information;
[0079] Step 4: Combining the generalized low-rank approximation method and the regularization term to construct an objective func...
Embodiment 2
[0119] Based on the classification model in the first embodiment, this embodiment provides a method for segmenting the macular lesion region of the fundus image, which adopts the classification model in the first embodiment, including:
[0120] Step 1: Classify the test image based on the classification model to obtain the foreground point and the background point of the test image;
[0121] Step 2: Take the area where the foreground point is located as the segmentation result.
[0122] Among them, step 1 specifically includes:
[0123] Grayscale the test image, scan the entire image with a k×k sliding window for sampling;
[0124] Using the optimal transformation matrix to reduce the dimensionality of the sample of the test image to obtain the optimal low-rank approximation matrix of the test image;
[0125] The optimal low-rank approximation matrix of the test image is used as the input of the SVM classifier to obtain the classification result.
[0126] If the test sample...
Embodiment 3
[0128] Based on the above image segmentation method, this embodiment provides a computer device for building a classification model for the segmentation of macular lesion regions in fundus images, including: a memory, a processor, and a computer program stored in the memory and operable on the processor , characterized in that, the processor implements the following steps when executing the program:
[0129] Receiving the user's selection of the fundus training image, performing grayscale processing on the training image to obtain a grayscale image; sampling the foreground and background of the grayscale image to obtain samples;
[0130] Obtaining a transformation matrix by using a generalized low-rank approximation method, performing dimensionality reduction processing on the sample based on the transformation matrix, and obtaining a low-rank approximation matrix of the sample;
[0131] Adding label information to the low-rank approximate matrix of the sample as supervision, ...
PUM
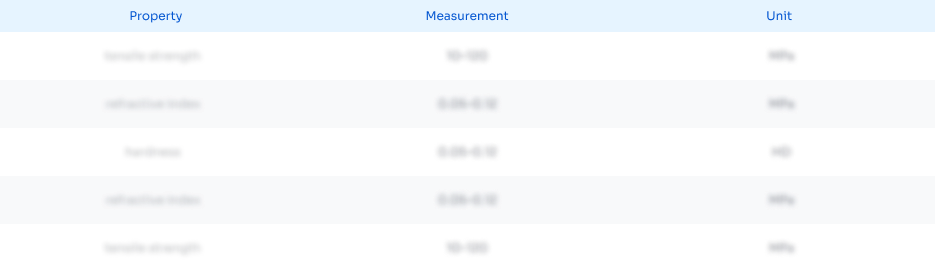
Abstract
Description
Claims
Application Information
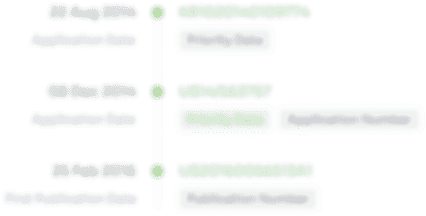
- Generate Ideas
- Intellectual Property
- Life Sciences
- Materials
- Tech Scout
- Unparalleled Data Quality
- Higher Quality Content
- 60% Fewer Hallucinations
Browse by: Latest US Patents, China's latest patents, Technical Efficacy Thesaurus, Application Domain, Technology Topic, Popular Technical Reports.
© 2025 PatSnap. All rights reserved.Legal|Privacy policy|Modern Slavery Act Transparency Statement|Sitemap|About US| Contact US: help@patsnap.com