GPR modeling based on kernel slow feature analysis and time delay estimation
A feature analysis and slow feature technology, applied in the field of GPR modeling, can solve problems such as long running time, impossibility of estimated delay, wrong conclusions, etc., to improve product quality, reduce production costs, and increase output.
- Summary
- Abstract
- Description
- Claims
- Application Information
AI Technical Summary
Problems solved by technology
Method used
Image
Examples
Embodiment Construction
[0020] Combine below image 3 Shown, the present invention is described in further detail:
[0021] Take the common chemical process—debutanizer process as an example. The experimental data come from the debutanizer process, and the butane concentration at the bottom of the debutanizer is predicted.
[0022] Step 1: Collect input and output data to form a historical training database.
[0023] Step 2: Standardize the training sample data and perform T for each input variable max +1 dimensional expansion. Among them, T max is the maximum delay.
[0024] Step 3: Determine the optimal time lag of each input variable by fuzzy curve analysis (FCA), defined as d 1 , d 2 ,...,d m . where m is the dimension of the sample. Described fuzzy curve analysis algorithm is:
[0025] FCA is used to select important input variables. By constructing the fuzzy logic between the input and output variables, the input variables that are important to the output variables are determined. ...
PUM
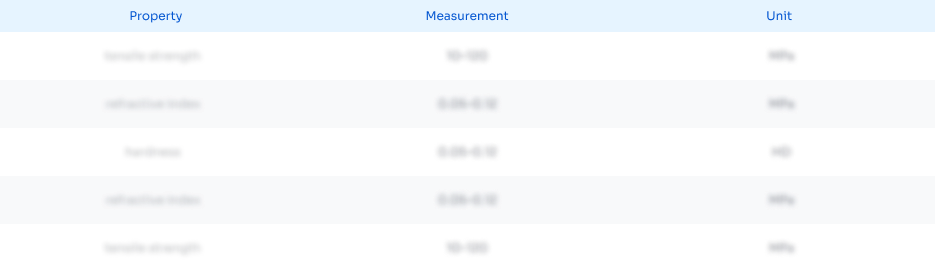
Abstract
Description
Claims
Application Information
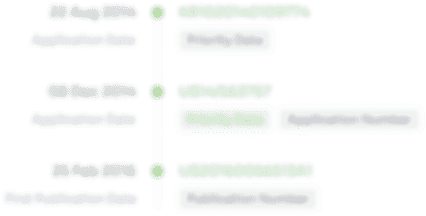
- R&D Engineer
- R&D Manager
- IP Professional
- Industry Leading Data Capabilities
- Powerful AI technology
- Patent DNA Extraction
Browse by: Latest US Patents, China's latest patents, Technical Efficacy Thesaurus, Application Domain, Technology Topic, Popular Technical Reports.
© 2024 PatSnap. All rights reserved.Legal|Privacy policy|Modern Slavery Act Transparency Statement|Sitemap|About US| Contact US: help@patsnap.com