RGBD image semantic segmentation method
A semantic segmentation and image technology, applied in the field of computer vision and pattern recognition, can solve problems such as not being able to integrate color images and depth images well, and not having global context information for learning images, so as to achieve high accuracy and improve accuracy Effect
- Summary
- Abstract
- Description
- Claims
- Application Information
AI Technical Summary
Problems solved by technology
Method used
Image
Examples
Embodiment Construction
[0057] The technical solutions of the present invention will be described in detail below in conjunction with the accompanying drawings and specific embodiments.
[0058] Such as figure 1 As shown, a kind of RGBD image semantic segmentation method provided by the present invention comprises the following steps:
[0059] S1. Collect data of training samples;
[0060] S2. Construct a configurable depth model, and input the data of training samples into the depth model to train the depth model;
[0061] S3. Obtain the color image and the corresponding depth image that need to be semantically segmented, analyze the color image and the depth image using the trained depth model, and predict the object category to which each pixel in the RGBD image belongs;
[0062] S4. According to the result of S3, form and output a predicted image semantic segmentation map;
[0063] Specifically, the S1 includes:
[0064] S101. Shoot the scene in the same direction at the same position through...
PUM
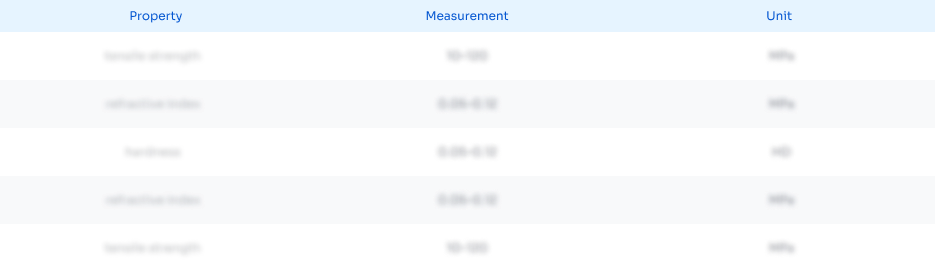
Abstract
Description
Claims
Application Information
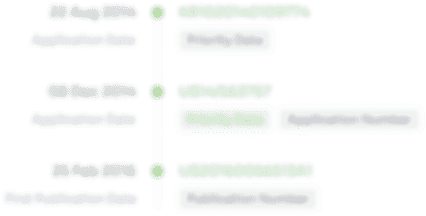
- R&D Engineer
- R&D Manager
- IP Professional
- Industry Leading Data Capabilities
- Powerful AI technology
- Patent DNA Extraction
Browse by: Latest US Patents, China's latest patents, Technical Efficacy Thesaurus, Application Domain, Technology Topic, Popular Technical Reports.
© 2024 PatSnap. All rights reserved.Legal|Privacy policy|Modern Slavery Act Transparency Statement|Sitemap|About US| Contact US: help@patsnap.com