Multi-scale small object detection method based on deep-learning hierarchical feature fusion
A technology of feature fusion and deep learning, applied to instruments, character and pattern recognition, computer components, etc., can solve problems such as size constraints, low detection accuracy, and difficulty in small object detection, and achieve real-time performance, recognition rate and The effect of improved positioning accuracy
- Summary
- Abstract
- Description
- Claims
- Application Information
AI Technical Summary
Problems solved by technology
Method used
Image
Examples
specific Embodiment approach 1
[0024] Specific Embodiment 1: The multi-scale small object detection method based on feature fusion between deep learning levels in this embodiment is characterized in that it includes:
[0025]Step 1. Use the pictures of the real scene database as training samples; each picture in the training samples has a pre-set marker position and category information; the marker position is used to indicate the position of the object to be recognized, and the category information is used to indicate the position of the object to be identified The kind of object.
[0026] Step 2. Initialize the candidate region generation network in the Resnet50 classification model trained by ImageNet, and train the candidate region generation network; during the training process, each time an input image is randomly selected from the data set as input, and the convolutional neural network is used to Generate a fusion feature map; the fusion feature map is generated by fusing multiple feature maps genera...
specific Embodiment approach 2
[0036] Specific embodiment 2: The difference between this embodiment and specific embodiment 1 is that in step 1, the training samples include: 1. Basic samples composed of MS COCO datasets; 2. Flipped samples obtained by flipping the basic samples left and right ; 3. The sample obtained by enlarging the basic sample and the flipped sample by a certain factor. The purpose of this embodiment is to make the training samples more comprehensive and rich, thereby making the recognition rate of the model higher.
[0037] Other steps and parameters are the same as in the first embodiment.
specific Embodiment approach 3
[0038] Embodiment 3: This embodiment differs from Embodiment 1 or Embodiment 2 in that in Step 2, the number of candidate regions generated by sliding convolution kernels on the fused feature map is 20,000. For each generated candidate region, if the overlap area between the candidate region and any marker position is greater than 0.55, it is considered as a positive sample, and if it is less than 0.35, it is considered as a negative sample. When calculating the loss function, 256 candidate regions are selected according to the scores of the candidate regions, and the ratio of positive and negative samples is 1:1. If the number of positive samples is less than 128, negative samples are used to fill them up. The final candidate area can be used (x 1 ,y 1 , x 2 ,y 2 ) means that x 1 ,y 1 Indicates the pixel coordinates of the upper left corner of the candidate area, x 2 ,y 2 Indicates the pixel coordinates of the upper right corner of the candidate area. Using this repre...
PUM
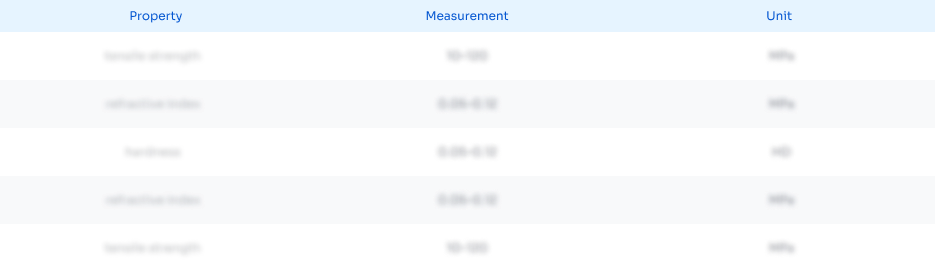
Abstract
Description
Claims
Application Information
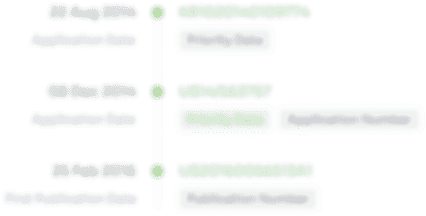
- R&D Engineer
- R&D Manager
- IP Professional
- Industry Leading Data Capabilities
- Powerful AI technology
- Patent DNA Extraction
Browse by: Latest US Patents, China's latest patents, Technical Efficacy Thesaurus, Application Domain, Technology Topic, Popular Technical Reports.
© 2024 PatSnap. All rights reserved.Legal|Privacy policy|Modern Slavery Act Transparency Statement|Sitemap|About US| Contact US: help@patsnap.com