Large-scale data abnormity detection method
A technology for large-scale data and anomaly detection, applied in electrical digital data processing, special data processing applications, genetic models, etc., can solve the problems of poor algorithm detection performance, easy to be affected by complex data, etc., to improve anomaly detection performance , The effect of reducing the workload of computing and reducing the amount of data
- Summary
- Abstract
- Description
- Claims
- Application Information
AI Technical Summary
Problems solved by technology
Method used
Image
Examples
Embodiment Construction
[0039] Such as figure 1 The anomaly detection method for large-scale data of the present invention includes:
[0040] A. Data preprocessing and feature extraction: Perform necessary data preprocessing on the original data, including data integration, data reduction and data cleaning, and then obtain preprocessed data sets and sample subsets. Then perform feature extraction on the preprocessed data, including:
[0041] A1. Data resampling: balance the samples of the preprocessed data through the preset ratio of positive and negative classes, and reduce the impact of negative samples on feature extraction;
[0042] A2. Calculation of information gain rate: Calculate the information gain rate of features through the data of multiple sample subsets, and sort the calculation results to form multiple feature sets; the method of calculating the information gain rate of features is:
[0043] Suppose the data set is D and the feature is A i (i=1,...,k), first calculate the entropy H...
PUM
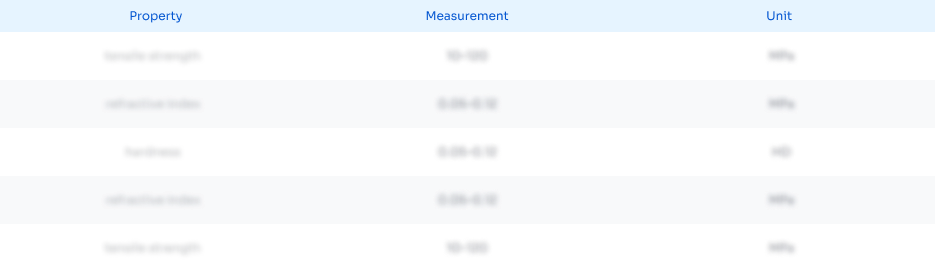
Abstract
Description
Claims
Application Information
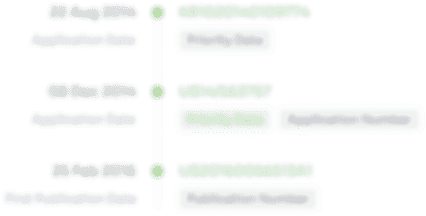
- R&D Engineer
- R&D Manager
- IP Professional
- Industry Leading Data Capabilities
- Powerful AI technology
- Patent DNA Extraction
Browse by: Latest US Patents, China's latest patents, Technical Efficacy Thesaurus, Application Domain, Technology Topic, Popular Technical Reports.
© 2024 PatSnap. All rights reserved.Legal|Privacy policy|Modern Slavery Act Transparency Statement|Sitemap|About US| Contact US: help@patsnap.com