Quick image classification method based on deep learning
A technology of deep learning and classification methods, applied in the fields of instruments, character and pattern recognition, computer parts, etc., can solve the problems of long training time, difficult training, low classification accuracy, etc., to speed up the classification speed, speed up the network convergence speed, The effect of ensuring classification accuracy
- Summary
- Abstract
- Description
- Claims
- Application Information
AI Technical Summary
Problems solved by technology
Method used
Image
Examples
Embodiment Construction
[0033] In order to better illustrate the technical solution of the present invention, the present invention will be further described below through an embodiment in conjunction with the accompanying drawings.
[0034] refer to figure 1 and figure 2 , a fast image classification method based on deep learning, the method includes four processes of deep convolutional neural network construction, data set preprocessing, network training and image classification testing.
[0035] The pictures in this implementation case are divided into 100 categories, and each category has 600 pictures. In each category of pictures, 500 pictures are randomly selected for training, and the remaining 100 pictures are used for testing. The image classification process is as follows figure 1 As shown, its structural framework is as follows figure 2 As shown, the operation steps include network construction, training process and testing process.
[0036] Described fast image classification metho...
PUM
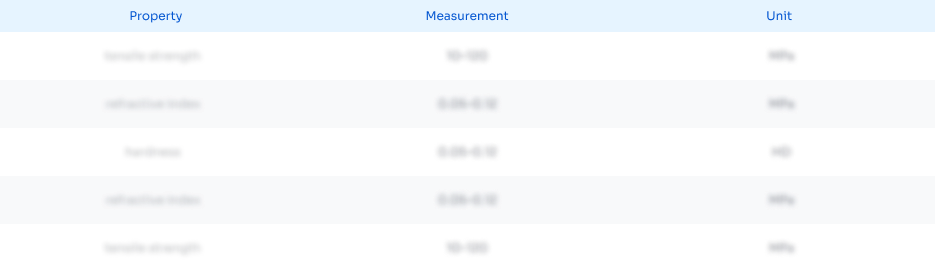
Abstract
Description
Claims
Application Information
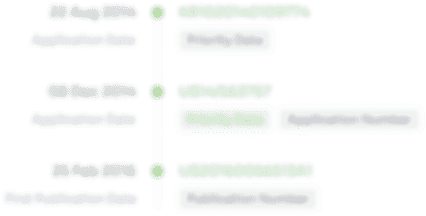
- R&D
- Intellectual Property
- Life Sciences
- Materials
- Tech Scout
- Unparalleled Data Quality
- Higher Quality Content
- 60% Fewer Hallucinations
Browse by: Latest US Patents, China's latest patents, Technical Efficacy Thesaurus, Application Domain, Technology Topic, Popular Technical Reports.
© 2025 PatSnap. All rights reserved.Legal|Privacy policy|Modern Slavery Act Transparency Statement|Sitemap|About US| Contact US: help@patsnap.com