Feature selection method based on optimal reconstruction
A feature selection method, the optimal technology, applied in the direction of complex mathematical operations, can solve the problems of not fully reflecting the distribution of the full amount of data, the effect of constraints, and high costs, so as to ensure the optimal expression performance, reduce the complexity of operations, and improve The effect of solving for efficiency
- Summary
- Abstract
- Description
- Claims
- Application Information
AI Technical Summary
Problems solved by technology
Method used
Image
Examples
Embodiment Construction
[0029] In order to make the above-mentioned features and advantages of the present invention more comprehensible, the following specific embodiments are described in detail in conjunction with the accompanying drawings.
[0030] In the application documents of the present invention, the symbol representation rules are: matrix representation as bold uppercase letters, vector representation as bold lowercase letters, and scalar representation as regular letters. Given matrix M=[m ij ], the i-th row vector and j-th column vector of the matrix are expressed as m i And m j . And vector The l p -The paradigm is defined as:
[0031]
[0032] matrix The l 2,1 -The paradigm is defined as:
[0033]
[0034] The present invention provides a feature selection method based on optimal reconstruction, such as figure 1 As shown, the steps include:
[0035] 1) Denote each original feature in the data set as d-dimensional data as a data matrix X, where d>1.
[0036] Assuming that the data set cont...
PUM
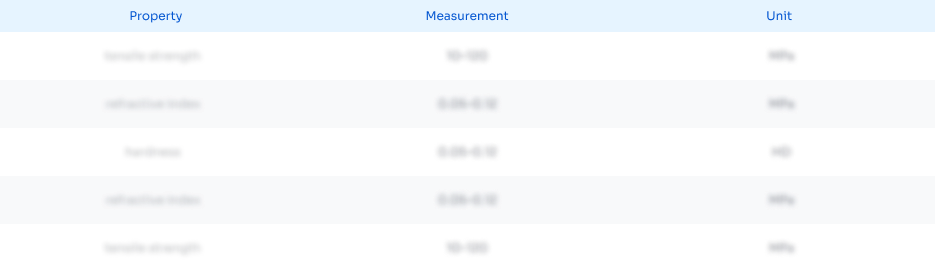
Abstract
Description
Claims
Application Information
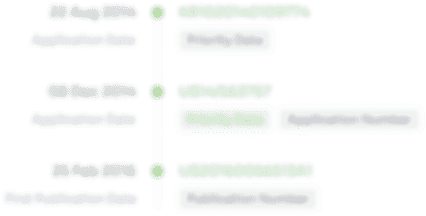
- R&D Engineer
- R&D Manager
- IP Professional
- Industry Leading Data Capabilities
- Powerful AI technology
- Patent DNA Extraction
Browse by: Latest US Patents, China's latest patents, Technical Efficacy Thesaurus, Application Domain, Technology Topic, Popular Technical Reports.
© 2024 PatSnap. All rights reserved.Legal|Privacy policy|Modern Slavery Act Transparency Statement|Sitemap|About US| Contact US: help@patsnap.com