A Fault Diagnosis Method of Bridge Crane Based on Bayesian Network
A bridge crane, Bayesian network technology, applied in computer parts, character and pattern recognition, instruments, etc., can solve the problems of no basis for the performance status of bridge cranes, time-consuming, low diagnostic efficiency, etc., and achieve strong reality Instructive effect
- Summary
- Abstract
- Description
- Claims
- Application Information
AI Technical Summary
Problems solved by technology
Method used
Image
Examples
Embodiment Construction
[0026] The embodiments of the present invention are described in detail below in conjunction with the accompanying drawings: the present embodiment is implemented on the premise of the technical solution of the present invention, and detailed implementation is provided, but the protection scope of the present invention is not limited to the following embodiments.
[0027] Such as figure 1 As shown, a bridge crane fault diagnosis method based on Bayesian network, first decomposes the bridge crane into modules, classifies the fault events that have occurred, and divides the fault levels and fault pivots according to the independent hierarchical pivot method, and obtains The fault tree model of the bridge crane establishes the Bayesian network structure of the bridge crane fault event; secondly, classifies the nodes in the Bayesian network structure to obtain the module state layer, fault reasoning layer and fault bottom event layer; thirdly, Collect the output signal of the modu...
PUM
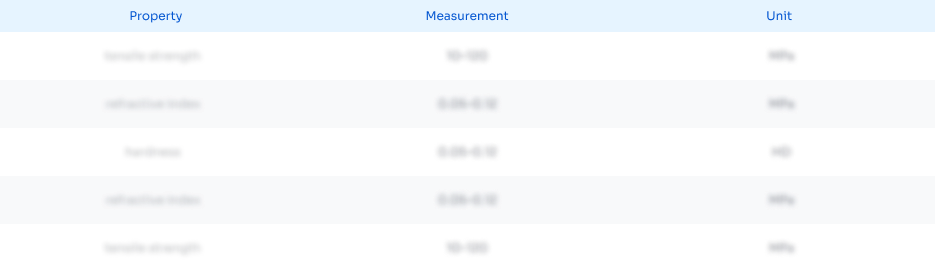
Abstract
Description
Claims
Application Information
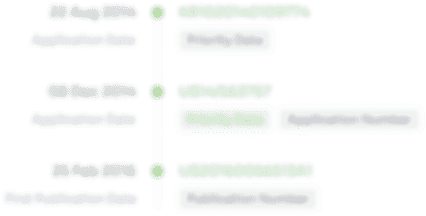
- R&D Engineer
- R&D Manager
- IP Professional
- Industry Leading Data Capabilities
- Powerful AI technology
- Patent DNA Extraction
Browse by: Latest US Patents, China's latest patents, Technical Efficacy Thesaurus, Application Domain, Technology Topic, Popular Technical Reports.
© 2024 PatSnap. All rights reserved.Legal|Privacy policy|Modern Slavery Act Transparency Statement|Sitemap|About US| Contact US: help@patsnap.com