3DCNN (three-dimensional convolutional neural network)-based high-spectral image space spectrum combined classification method
A technology of hyperspectral image and classification method, which is applied in 3DCNN-based space-spectrum joint classification of hyperspectral image and classification field of hyperspectral image, which can solve the problems of loss of spectral information, influence on accuracy, and large amount of calculation for dimension reduction processing, etc., to achieve The effect of expanding the application range and improving the classification accuracy
- Summary
- Abstract
- Description
- Claims
- Application Information
AI Technical Summary
Problems solved by technology
Method used
Image
Examples
Embodiment Construction
[0019] Now in conjunction with embodiment, accompanying drawing, the present invention will be further described:
[0020] Step 1: Input hyperspectral image data, according to the formula Normalize the data. where x ijs Represents a pixel in the hyperspectral image, i and j respectively represent the coordinate position of the pixel in the hyperspectral image, s represents the spectral segment of the hyperspectral image, and the existing hyperspectral image generally contains 100-240 spectral segments , x ··smax 、x ··smin Respectively represent the maximum and minimum values of the three-dimensional hyperspectral image in the s-band.
[0021] Step 2 Extract the original spatial spectral features, and extract the data block P within a certain neighborhood centered on the pixel to be classified from the hyperspectral image n×n×L , n represents the size of the neighborhood block, generally 5 or 7, L represents the total number of spectral segments, data block P n×n×L is ...
PUM
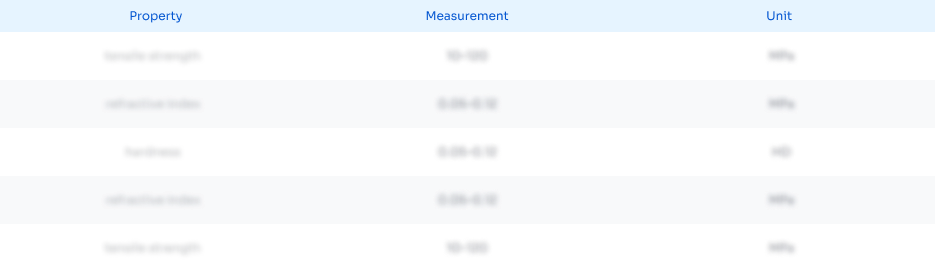
Abstract
Description
Claims
Application Information
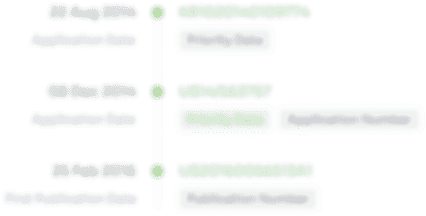
- R&D Engineer
- R&D Manager
- IP Professional
- Industry Leading Data Capabilities
- Powerful AI technology
- Patent DNA Extraction
Browse by: Latest US Patents, China's latest patents, Technical Efficacy Thesaurus, Application Domain, Technology Topic, Popular Technical Reports.
© 2024 PatSnap. All rights reserved.Legal|Privacy policy|Modern Slavery Act Transparency Statement|Sitemap|About US| Contact US: help@patsnap.com