Hierarchical clustering-based system steady state detection algorithm
A technology of hierarchical clustering and detection algorithm, applied in computing, computer parts, instruments, etc., can solve the problems of poor universality, strong dependence on process objects, weak applicability, etc., to achieve strong applicability and avoid the amount of calculation. dramatic effect
- Summary
- Abstract
- Description
- Claims
- Application Information
AI Technical Summary
Problems solved by technology
Method used
Image
Examples
Embodiment 1
[0067] Embodiment 1 is aimed at a second-order single-input single-output system, and the input and output after simulation processing by the method of the present invention are as follows: Figure 4 As shown, the simulation time length is 100s, the sampling interval is 0.1s, Figure 4 (a) represents the input variable curve, Figure 4 (b) shows the output variable curve.
[0068] Noise is not added in the variable of embodiment 1, which can be obtained from Figure 4 It can be seen from the curve that the system is in a steady state at the initial stage (t=0~300), and at t=300 the input generates a ramp adjustment, and the system enters a transitional state. When the ramp signal ends after t=600, the output also tends to Stabilization The final system enters another steady state. Since no noise is added, the degree of aggregation of the system state is very high in the steady state. Here, instead of using the threshold selection method of the above algorithm, a small thres...
Embodiment 2
[0073] Embodiment 2 adds noise for simulation, and further explains the threshold selection process based on the single-input-single-out system process used in Embodiment 1. The input and output of the simulation system with noise added is as follows Figure 7 as shown, Figure 7 (a) represents the input variable curve, Figure 7 (b) represents the output variable curve:
[0074] Figure 7 It can be seen from the figure that the system is in a steady state within the interval of time t=0~300, and then the input u produces a ramp change, and after a period of transition state, the system enters another steady state. In the state space of the whole process, the state points of the two steady states are highly aggregated, while the transition state points are scattered. The selection of the threshold is enumerated from large to small. As the clustering threshold shrinks, the degree of concentration within each class becomes higher and higher. When the jth step is reached, if ...
Embodiment 3
[0078] Embodiment 3 is applied to the historical data generated in the actual process, and the boiler data of a 60MW unit of a certain power plant is used for data experimentation. The data mainly includes: boiler load command, power generation, coal intake, air intake, steam water temperature and pressure at each measurement point, etc., a total of 180 dimensions. Select 10,000 points of data and use the above clustering algorithm for steady-state detection. Changes in the main variables during this period are as follows: Figure 9 Shown:
[0079] From Figure 9 It can be seen from the figure that in the time period studied, there were two major adjustments in the system load, and roughly three periods of steady-state data were generated within the interval. Both the main steam temperature and the main steam pressure fluctuate greatly, and the fluctuation of the system cannot even be seen from the steam temperature curve. Next, the state points composed of all the variabl...
PUM
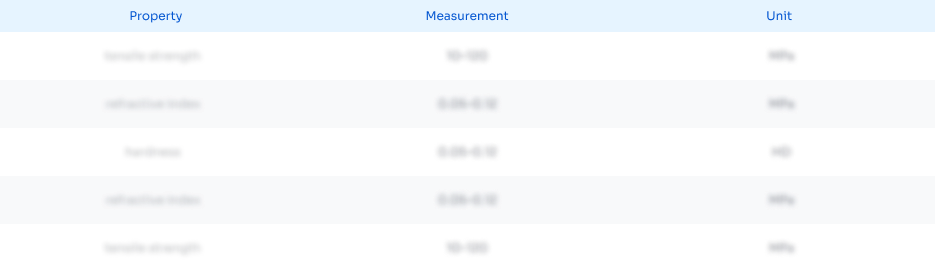
Abstract
Description
Claims
Application Information
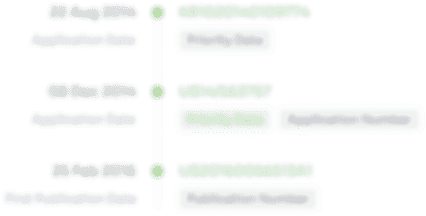
- R&D Engineer
- R&D Manager
- IP Professional
- Industry Leading Data Capabilities
- Powerful AI technology
- Patent DNA Extraction
Browse by: Latest US Patents, China's latest patents, Technical Efficacy Thesaurus, Application Domain, Technology Topic, Popular Technical Reports.
© 2024 PatSnap. All rights reserved.Legal|Privacy policy|Modern Slavery Act Transparency Statement|Sitemap|About US| Contact US: help@patsnap.com