Abnormal behavior detection method based on time-space Laplacian Eigenmaps learning
A Laplacian and feature mapping technology, applied in instruments, computing, character and pattern recognition, etc., can solve problems such as complex background, huge time overhead and computational complexity, and inability to track and detect each individual
- Summary
- Abstract
- Description
- Claims
- Application Information
AI Technical Summary
Problems solved by technology
Method used
Image
Examples
Embodiment Construction
[0043] Implementation language: Matlab
[0044] Hardware platform: Inteli32120+4GDDRRAM
[0045] The method of the present invention is verified by an intuitive and effective algorithm on Matlab.
[0046] The algorithm of the present invention, the random forest method and the template matching method were verified experiments on the Caviar database. The experimental results are as follows image 3 As shown, the random forest method (such as image 3 A) and template matching method (such as image 3 (Shown in B) are sensitive to light changes, causing false detections. The present invention has strong robustness to illumination transformation (such as image 3 C), which reduces the false detection rate to a certain extent and improves the accuracy.
[0047] The actual monitoring video is used to compare the template matching method with the method of the present invention, and the experimental results are as follows Figure 4 As shown, the template matching method can only roughly det...
PUM
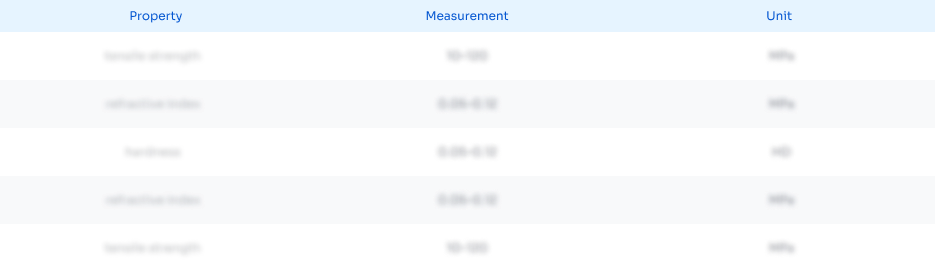
Abstract
Description
Claims
Application Information
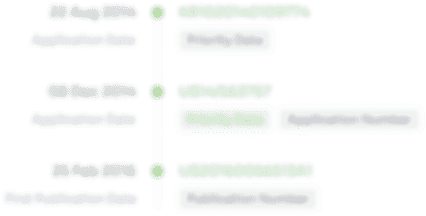
- R&D Engineer
- R&D Manager
- IP Professional
- Industry Leading Data Capabilities
- Powerful AI technology
- Patent DNA Extraction
Browse by: Latest US Patents, China's latest patents, Technical Efficacy Thesaurus, Application Domain, Technology Topic.
© 2024 PatSnap. All rights reserved.Legal|Privacy policy|Modern Slavery Act Transparency Statement|Sitemap