Fault diagnosis method based on metric learning and time sequence during industrial process
A metric learning and time-series technology, applied to instruments, electrical testing/monitoring, control/regulation systems, etc., can solve problems such as high system cost, difficulty in distinguishing fault types, and difficulty in online diagnosis, so as to reduce the amount of calculation and improve promotion performance, design and ease of operation
- Summary
- Abstract
- Description
- Claims
- Application Information
AI Technical Summary
Problems solved by technology
Method used
Image
Examples
specific Embodiment approach 1
[0023] Embodiment 1: A fault diagnosis method based on metric learning and time series in an industrial process of this embodiment is specifically prepared according to the following steps:
[0024] Step 1: Analyze the historical monitoring data, and divide the system failures into n types, that is, n subclasses, according to the different causes of the system failures;
[0025] Step 2: Collect the standard data under the normal operating conditions of the system and the operating states of n fault operating conditions as training samples;
[0026] Step 3: Preprocessing the training samples by using a time series method; wherein, the preprocessing method refers to using wavelet transform to process the training samples;
[0027] Step 4: Perform metric learning on the preprocessed training samples, and generate a metric matrix of the system as a standard for measuring the similarity of the samples, that is, generate a metric matrix A of the system;
[0028] Step 5. After the r...
specific Embodiment approach 2
[0039] Embodiment 2: The difference between this embodiment and Embodiment 1 is that the metric learning in step 4 is specifically: metric learning focuses on analyzing the differences between data of different fault types, and obtains a metric matrix through training samples, which is used in online fault diagnosis. There are obvious advantages; metric learning realizes the distinction of fault types by obtaining a distance matrix that can accurately describe the similarity of samples;
[0040] (1) Obtain the distance M between all training samples and real-time monitoring samples through metric learning A Expressed as:
[0041] M A = ( x - y ) T A ( x - y ...
specific Embodiment approach 3
[0049] Embodiment 3: The difference between this embodiment and Embodiment 1 or 2 is that a specific iterative process for calculating the metric matrix A by selecting an iterative method based on gradient descent is described as follows:
[0050] (1) Select a pair of constraints (i, j);
[0051] (2) Calculate p=(x i -x j ) T A k (x i -x j );
[0052] (3) When (i,j)∈S, take δ=1, otherwise take δ=-1;
[0053] (4) Calculation a = m i n ( λ i j , δ 2 ( 1 p - γ ξ c ( i , j ...
PUM
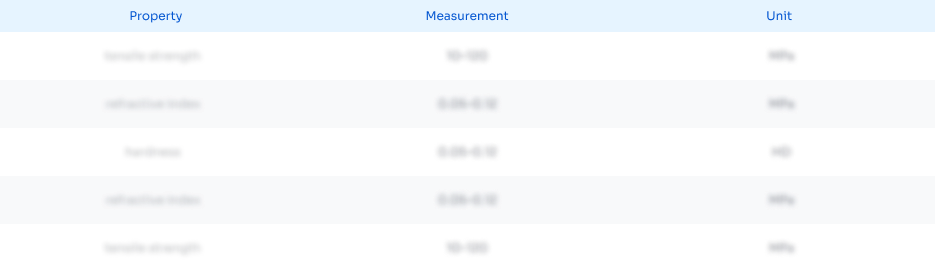
Abstract
Description
Claims
Application Information
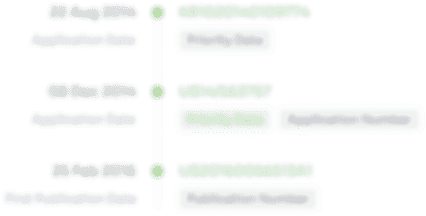
- Generate Ideas
- Intellectual Property
- Life Sciences
- Materials
- Tech Scout
- Unparalleled Data Quality
- Higher Quality Content
- 60% Fewer Hallucinations
Browse by: Latest US Patents, China's latest patents, Technical Efficacy Thesaurus, Application Domain, Technology Topic, Popular Technical Reports.
© 2025 PatSnap. All rights reserved.Legal|Privacy policy|Modern Slavery Act Transparency Statement|Sitemap|About US| Contact US: help@patsnap.com