Artificial-neural-network-based inverse design method for aircraft airfoils/wings
An artificial neural network and anti-design technology, applied in computing, special data processing applications, instruments, etc., can solve problems such as different intelligent algorithms and unsatisfactory results, and achieve accurate results
- Summary
- Abstract
- Description
- Claims
- Application Information
AI Technical Summary
Problems solved by technology
Method used
Image
Examples
Embodiment 1
[0031] Design requirements: Under a cruise Mach number Ma=0.785, the design state is an airfoil with the following parameters:
[0032] Design Mach number Ma=0.71
[0033]Design angle of attack á=2.53
[0034] Reynolds number Re=23,000,000
[0035] First create the airfoil database. In terms of shape structure, the airfoil is relatively simple. In this example, the airfoil database contains 208 reconstructed airfoils. Each airfoil in the database must have good aerodynamic performance; and the geometric configuration of the airfoil must have as large a span as possible, that is, there must be obvious shape differences between the airfoils. Only in this way can the efficiency and integrity of the airfoil database be guaranteed. Table 1 and Table 2 summarize the span of airfoil movement parameters and shape parameters, respectively.
[0036] Table 1 Summary of airfoil aerodynamic parameters
[0037]
[0038] Table 2 Summary of airfoil shape parameters
[0039] ...
Embodiment 2
[0047] Design requirements: The designed lift-to-drag ratio is 25.8, the lift coefficient is 0.5, the form drag coefficient is 0.0105, the induced drag coefficient is 0.009, the shock wave drag coefficient is 0.00017, and the moment coefficient is 0.23; the wing lift coefficient is 0.43, and the drag coefficient is Wing profile with a moment coefficient of 0.0095 and -0.13.
[0048] The main aerodynamic parameters (wing lift-to-drag ratio and lift coefficient) are proposed by the designer, and the rest of the secondary aerodynamic parameters are given by interpolation method with reference to the main aerodynamic parameters and the 214 sets of aerodynamic input ranges in the database, as shown in Table 5.
[0049] Table 5 Summary of wing aerodynamic parameters
[0050] .
[0051] First use the SOM neural network to classify the wing data in the database according to the aerodynamic performance, take out a set of data corresponding to the target aerodynamic input, and select...
PUM
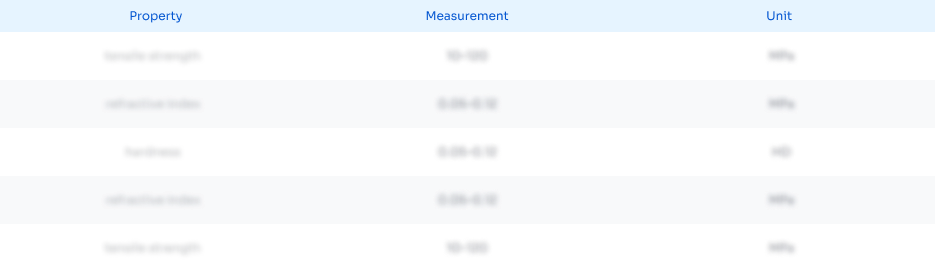
Abstract
Description
Claims
Application Information
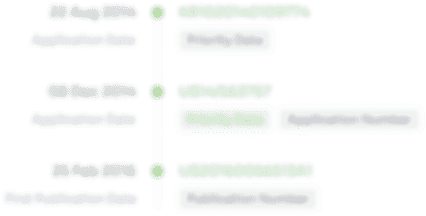
- R&D Engineer
- R&D Manager
- IP Professional
- Industry Leading Data Capabilities
- Powerful AI technology
- Patent DNA Extraction
Browse by: Latest US Patents, China's latest patents, Technical Efficacy Thesaurus, Application Domain, Technology Topic, Popular Technical Reports.
© 2024 PatSnap. All rights reserved.Legal|Privacy policy|Modern Slavery Act Transparency Statement|Sitemap|About US| Contact US: help@patsnap.com