Internet flow distinguishing method
A technology for Internet traffic and network traffic, applied in the field of Internet traffic classification, can solve problems such as high function density, difficult traffic samples, and failure to complete classification tasks, and achieve the effect of ensuring timeliness
- Summary
- Abstract
- Description
- Claims
- Application Information
AI Technical Summary
Problems solved by technology
Method used
Image
Examples
Embodiment Construction
[0080] Refer to the attached figure 1 , is the network topology diagram of the network environment deployed by the Internet traffic differentiation method with online intelligent identification capabilities, such as figure 1 shown. In the measured network, choose to deploy modules based on accurate application marking on a small number of network nodes. The egress, or network boundary, is mirrored to an FPGA-based network traffic forwarder. This forwarder forwards all web traffic to servers running semi-supervised clustering and supervised learning classification. The latter feeds labeled data into the training classifier module and unlabeled traffic into the online classification module.
[0081] According to the above content, some technical problems are further defined. One purpose is the design of the online real-time classification module of network traffic, which solves the problem that the existing technology cannot realize online real-time classification of network ...
PUM
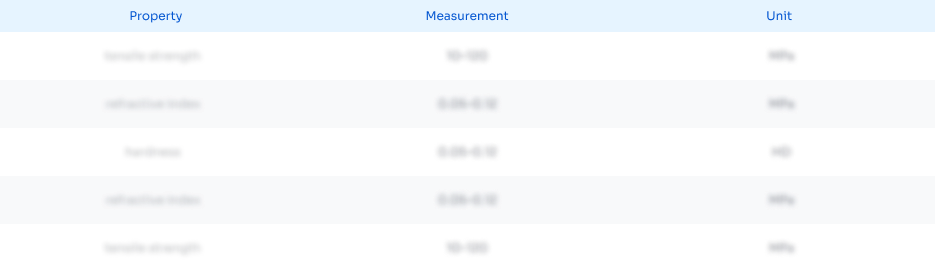
Abstract
Description
Claims
Application Information
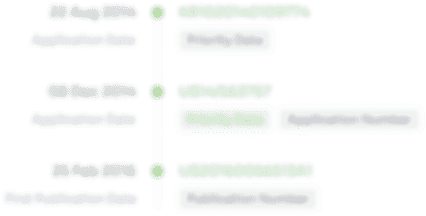
- R&D Engineer
- R&D Manager
- IP Professional
- Industry Leading Data Capabilities
- Powerful AI technology
- Patent DNA Extraction
Browse by: Latest US Patents, China's latest patents, Technical Efficacy Thesaurus, Application Domain, Technology Topic, Popular Technical Reports.
© 2024 PatSnap. All rights reserved.Legal|Privacy policy|Modern Slavery Act Transparency Statement|Sitemap|About US| Contact US: help@patsnap.com