Intelligence type retrieval dialogue method based on pre-training and attention interaction network
A pre-training and attention technology, applied in the fields of deep learning and natural language processing, can solve the problem of the decline of separation coding accuracy, and achieve the effect of improving the ability of accurate retrieval response, enhancing the ability of representation, and improving the retrieval speed.
- Summary
- Abstract
- Description
- Claims
- Application Information
AI Technical Summary
Problems solved by technology
Method used
Image
Examples
Embodiment 1
[0091] The knowledge-based retrieval dialogue method based on pre-training and attention interaction network in this embodiment is implemented on a role-based dialogue corpus (Persona-Chat). The implementation process includes a domain-adaptive pre-training phase and a fine-tuning training phase.
[0092] figure 1 , figure 2 is an explanatory diagram of the field adaptability pre-training stage during the implementation of the present invention, such as figure 1 , figure 2 As shown, the basic steps of domain-adaptive pre-training implemented on the Persona-Chat corpus are as follows:
[0093] S1. The pre-trained language model of this embodiment selects the basic, case-insensitive BERT model proposed by Google. The BERT model is a neural network including a 12-layer, 768-dimensional, 12 self-attention head, and 110M parameters. Structure; the domain adaptive pre-training hyperparameters are set as follows: the training batch size is 20, the dropout probability is 0.2, th...
Embodiment 2
[0159] The knowledge-based retrieval dialogue method based on pre-training and attention interaction network of the present invention is implemented on a document-based dialogue corpus (CMUDoG). The implementation process includes a domain-adaptive pre-training phase and a fine-tuning training phase.
[0160] figure 1 , figure 2 is an explanatory diagram of the field adaptability pre-training stage in the implementation process of the present invention, such as figure 1 , figure 2 As shown, the basic steps of domain-adaptive pre-training implemented on the CMUDoG corpus are as follows:
[0161] S1. The pre-trained language model of this embodiment selects the basic, case-insensitive BERT model proposed by Google, and the BERT model is a neural network including a 12-layer, 768-dimensional, 12 self-attention head, and 110M parameters Structure; the domain adaptive pre-training hyperparameters are set as follows: the training batch size is 10, the dropout probability is 0....
Embodiment 3
[0217] The knowledge-based retrieval dialogue method based on pre-training and attention interaction network of the present invention is implemented on a document-based dialogue corpus (Persona-Chat). The implementation process includes a domain-adaptive pre-training phase and a fine-tuning training phase.
[0218] figure 1 , figure 2 is an explanatory diagram of the field adaptability pre-training stage in the implementation process of the present invention, such as figure 1 , figure 2 As shown, the basic steps of domain-adaptive pre-training implemented on the Persona-Chat corpus are as follows:
[0219] S1. The pre-trained language model of this embodiment selects the ALBERT model proposed by Google with a faster training speed. The ALBERT model includes a 12-layer, 128-dimensional embedding layer, 128-dimensional hidden layer, 12 self-attention heads, 10M The neural network structure of the parameters; the domain adaptive pre-training hyperparameters are set as follo...
PUM
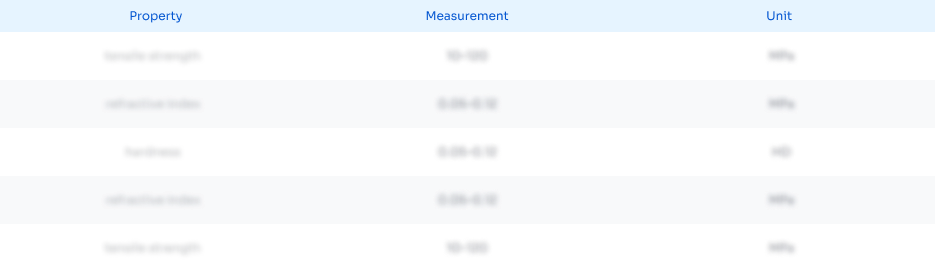
Abstract
Description
Claims
Application Information
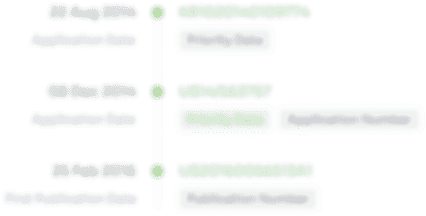
- R&D Engineer
- R&D Manager
- IP Professional
- Industry Leading Data Capabilities
- Powerful AI technology
- Patent DNA Extraction
Browse by: Latest US Patents, China's latest patents, Technical Efficacy Thesaurus, Application Domain, Technology Topic, Popular Technical Reports.
© 2024 PatSnap. All rights reserved.Legal|Privacy policy|Modern Slavery Act Transparency Statement|Sitemap|About US| Contact US: help@patsnap.com