Multi-device integrated calligraphy teaching system
A teaching system and multi-equipment technology, applied in the direction of electronically operated teaching aids, teaching aids, educational appliances, etc., can solve the problems of single evaluation standard, inability to intuitively find, and copying methods detached.
- Summary
- Abstract
- Description
- Claims
- Application Information
AI Technical Summary
Problems solved by technology
Method used
Image
Examples
Embodiment 1
[0032] A calligraphy teaching system integrating multiple devices, a calligraphy teaching system integrating multiple devices, comprising a calligraphy practice module, a surface electromyographic signal acquisition, a practice evaluation module and a calligraphy tutorial module, characterized in that: the calligraphy practice module is composed of writing The posture correction function, the writing strength correction function and the word cutting function are composed. The surface EMG signal acquisition is composed of the feature extraction of the surface EMG signal, the classification of the surface EMG signal, the muscle strength estimation of the surface EMG signal and the posture recognition of the image. The exercise evaluation module consists of offline handwritten Chinese character recognition and handwritten Chinese character image comparison. The electromyographic signal (EMG) is a series of motor unit action potentials generated in the process of muscle excitation ...
Embodiment 2
[0033]Embodiment 2: The difference between this embodiment and Embodiment 1 is that the feature extraction of the surface EMG signal adopts PCA-based multi-channel EMG information fusion. During the muscle exertion process, the contact loosening and relative displacement between the array surface electrodes and the skin will introduce corresponding noise, resulting in pseudo-muscle activation and abnormal overall activation intensity during the resting period. Using the preprocessing method of PCA, the signal denoising and enhancement of the channel which is seriously polluted by noise can be well achieved. The classification of the surface EMG signals was performed using LSTM. The sampling frequency of the EMG signal is 1000Hz, and the writing process may be as long as tens of seconds, which leads to a long sequence of EMG signals. In order to solve the problem of gradient disappearance and gradient explosion in the long sequence training process, LSTML is used. Perform clas...
Embodiment 3
[0034] Embodiment 3: The difference between this implementation and Embodiment 1 is that the offline handwritten Chinese character recognition adopts the DBN+CNN technology. Offline handwritten Chinese characters have many near-character shapes, and it is difficult to extract features and easy to identify inaccurately. And in the project application scenario, it is necessary to recognize Chinese characters of various writing levels. A fusion model of convolutional neural network CNN and deep belief network DBN is now used. Using CNN to automatically extract image features and DBN's advantages of continuously weakening the errors and secondary information of each layer of network, a fusion comparison strategy is adopted to extract a classification result as much as possible to improve the recognition ability. The choice of the handwritten Chinese character image comparison technique is to use a twin neural network. The Siamese neural network has two inputs, and the two inputs...
PUM
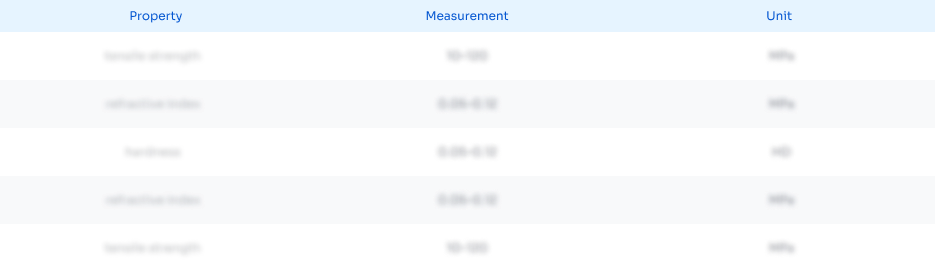
Abstract
Description
Claims
Application Information
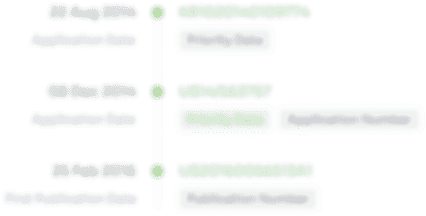
- R&D Engineer
- R&D Manager
- IP Professional
- Industry Leading Data Capabilities
- Powerful AI technology
- Patent DNA Extraction
Browse by: Latest US Patents, China's latest patents, Technical Efficacy Thesaurus, Application Domain, Technology Topic, Popular Technical Reports.
© 2024 PatSnap. All rights reserved.Legal|Privacy policy|Modern Slavery Act Transparency Statement|Sitemap|About US| Contact US: help@patsnap.com