Fan fault transferable diagnosis method based on data enhancement and capsule neural network
A technology of neural network and diagnosis method, which is applied in the field of transferable diagnosis of fan faults based on data enhancement and capsule neural network, can solve a large amount of industrial field data, cannot send relevant personnel to inspect the running status of fans frequently, and has limited fault data, etc. It can simplify the calculation process, improve the fault diagnosis performance, and improve the versatility.
- Summary
- Abstract
- Description
- Claims
- Application Information
AI Technical Summary
Problems solved by technology
Method used
Image
Examples
Embodiment Construction
[0055] The present invention will be described in detail below according to the accompanying drawings and preferred embodiments, and the purpose and effects of the present invention will become clearer.
[0056] The method for diagnosing fan faults based on data enhancement and capsule neural network can be migrated according to the present invention, and the specific flow chart is as follows: figure 1 shown, including the following steps:
[0057] S1: Preprocess the collected fan vibration signal data, and detect and eliminate abnormal values in the vibration signal data according to the Laida criterion.
[0058] The formula for the Laida criterion is as follows:
[0059] (1)
[0060] in, x t for the first t a fan vibration signal, represents the average value of the vibration signal of this segment, is the standard deviation of the segment signal, n Indicates the total number of sampling points in this segment of the signal; when the data meets the Laida crit...
PUM
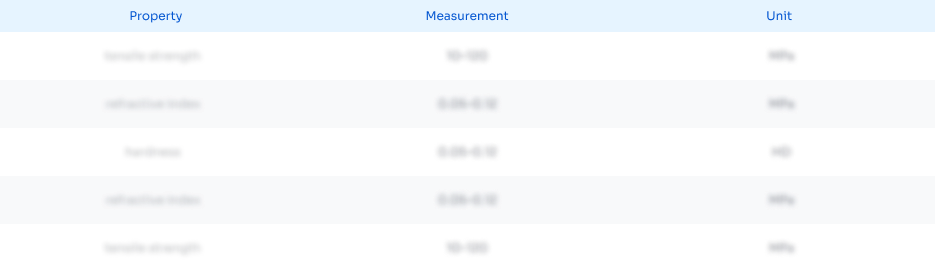
Abstract
Description
Claims
Application Information
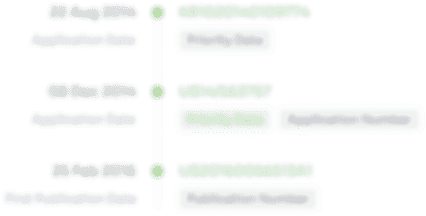
- Generate Ideas
- Intellectual Property
- Life Sciences
- Materials
- Tech Scout
- Unparalleled Data Quality
- Higher Quality Content
- 60% Fewer Hallucinations
Browse by: Latest US Patents, China's latest patents, Technical Efficacy Thesaurus, Application Domain, Technology Topic, Popular Technical Reports.
© 2025 PatSnap. All rights reserved.Legal|Privacy policy|Modern Slavery Act Transparency Statement|Sitemap|About US| Contact US: help@patsnap.com