Cross-supervised model training method, image segmentation method and related equipment
A technology for model training and image segmentation, applied in the field of image processing, can solve the problems of inability to guarantee the accuracy of model operation and difficulty in labeling, and achieve the effect of reducing labeling and improving accuracy.
- Summary
- Abstract
- Description
- Claims
- Application Information
AI Technical Summary
Problems solved by technology
Method used
Image
Examples
Embodiment Construction
[0027] In order to make the purpose, technical scheme and effect of the present invention clearer and clearer, the present invention will be described in further detail below with reference to the accompanying drawings and examples.
[0028] The present application provides a cross-supervised model training method. By establishing a twin network, the output results of the two networks are respectively used as the training labels of the other network for training. Using the cross-supervised method can reduce the labeling of initial sample images. Improve the accuracy of model operations.
[0029] see figure 1 , figure 1 It is a schematic flowchart of an embodiment of the model training method of the present application. It should be noted that if there are substantially the same results, this embodiment does not figure 1 The sequence of processes shown is limited. like figure 1 As shown, this embodiment includes:
[0030] S110: Acquire a sample image.
[0031] S130: Inpu...
PUM
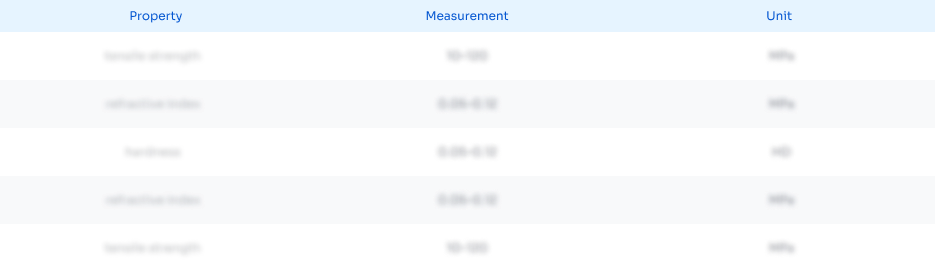
Abstract
Description
Claims
Application Information
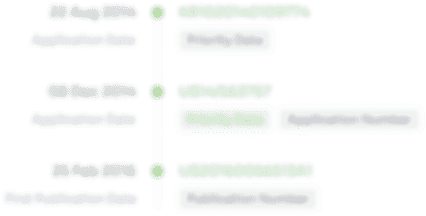
- R&D Engineer
- R&D Manager
- IP Professional
- Industry Leading Data Capabilities
- Powerful AI technology
- Patent DNA Extraction
Browse by: Latest US Patents, China's latest patents, Technical Efficacy Thesaurus, Application Domain, Technology Topic, Popular Technical Reports.
© 2024 PatSnap. All rights reserved.Legal|Privacy policy|Modern Slavery Act Transparency Statement|Sitemap|About US| Contact US: help@patsnap.com