Semi-supervised learning method for carrying out cell nucleus segmentation on histopathological image
A histopathology, cell nucleus technology, applied in the field of semi-supervised learning, can solve the problems of inconsistent staining operation, difficult to eliminate the difference of image content, difference of image style, etc.
- Summary
- Abstract
- Description
- Claims
- Application Information
AI Technical Summary
Problems solved by technology
Method used
Image
Examples
Embodiment Construction
[0012] specific implementation
[0013] The specific process of implementing this method is as follows: figure 1 As shown, the semi-supervised learning cell nucleus segmentation method provided by the present invention includes the following steps:
[0014] 1. Obtain a large amount of unlabeled data, that is, histopathological images stained with hematoxylin and eosin (full-field digital sections);
[0015] 2. Process the obtained full-field digital slices, including dividing the large-sized full-field digital slices into smaller images, such as 1000×1000; blank picture;
[0016] 3. Using non-negative matrix factorization with sparse constraints to separate unlabeled hematoxylin and eosin-stained histopathological images;
[0017] 4. Select an anchor image, then randomly select two positive sample images for the anchor image, and replace the hematoxylin stain in the positive sample image with the hematoxylin stain in the anchor image, thereby constructing a A set of positi...
PUM
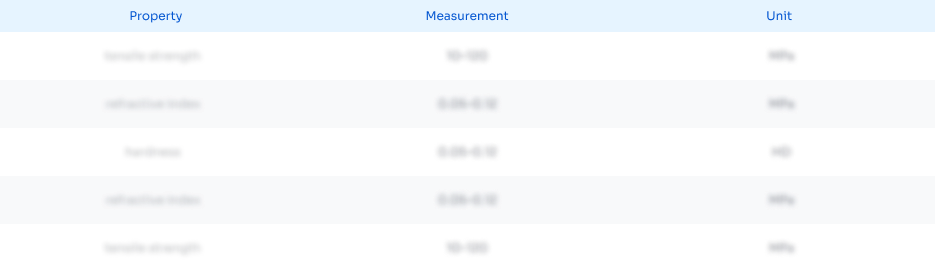
Abstract
Description
Claims
Application Information
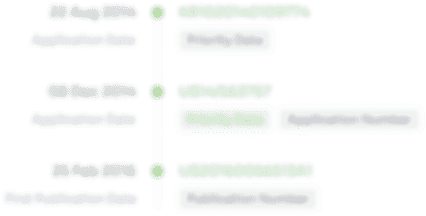
- R&D Engineer
- R&D Manager
- IP Professional
- Industry Leading Data Capabilities
- Powerful AI technology
- Patent DNA Extraction
Browse by: Latest US Patents, China's latest patents, Technical Efficacy Thesaurus, Application Domain, Technology Topic, Popular Technical Reports.
© 2024 PatSnap. All rights reserved.Legal|Privacy policy|Modern Slavery Act Transparency Statement|Sitemap|About US| Contact US: help@patsnap.com