Electrocardiosignal noise reduction method based on variational self-coding and Pixel CNN model
An electrocardiographic signal and self-encoding technology, applied in neural learning methods, character and pattern recognition, biological neural network models, etc., can solve the problems of signal distortion, loss of key signal information, and large amount of calculation, so as to reduce reconstruction errors. , reduce the computational cost, and facilitate the extraction effect
- Summary
- Abstract
- Description
- Claims
- Application Information
AI Technical Summary
Problems solved by technology
Method used
Image
Examples
Embodiment 1
[0045] Step b) includes the following steps:
[0046] by formula Calculate the normalized clean ECG sample data where coef is the coefficient, ub is the upper limit of the normalized range required for the signal, lb is the lower limit of the normalized range required for the signal, s max is the clean ECG signal sample data S i the maximum value of s min is the clean ECG signal sample data S i the minimum value of s mid is the clean ECG signal sample data S i the median value of , mid is the middle value of the range required for signal normalization,
Embodiment 2
[0048] In step c), three noise signals MA, BW, and EM in the MIT-BIH noise stress test database are selected as noise signals, and each noise signal in the three noise signals MA, BW, and EM has 650,000 sampling points. The three noise signals BW and EM are sampled at random starting points respectively, and L noise sample data are obtained.
Embodiment 3
[0050] In step d), the noise signal sample data N is i Add clean ECG signal sample data S at 5dB intensity i middle.
PUM
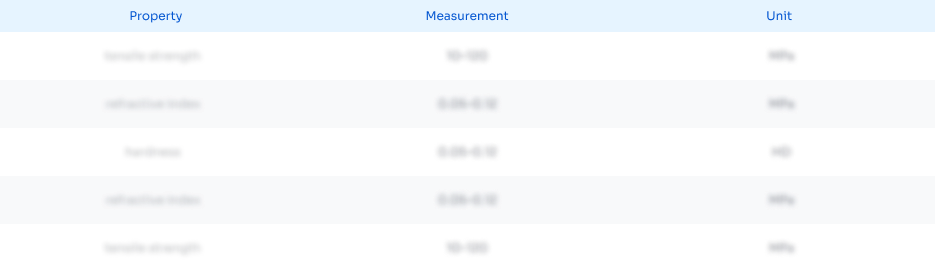
Abstract
Description
Claims
Application Information
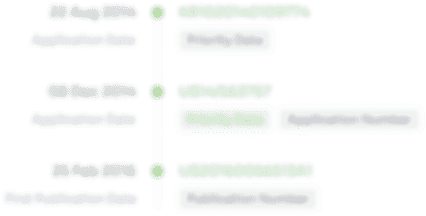
- Generate Ideas
- Intellectual Property
- Life Sciences
- Materials
- Tech Scout
- Unparalleled Data Quality
- Higher Quality Content
- 60% Fewer Hallucinations
Browse by: Latest US Patents, China's latest patents, Technical Efficacy Thesaurus, Application Domain, Technology Topic, Popular Technical Reports.
© 2025 PatSnap. All rights reserved.Legal|Privacy policy|Modern Slavery Act Transparency Statement|Sitemap|About US| Contact US: help@patsnap.com