Federal learning system and federal learning training method for smart city Internet of Things belief fusion
A learning system, learning and training technology, applied in machine learning, transmission systems, advanced technologies, etc., can solve problems such as client offset, unstable convergence process, local model deviation from global model, etc., to protect data privacy, improve model Effects of Convergence Performance
- Summary
- Abstract
- Description
- Claims
- Application Information
AI Technical Summary
Problems solved by technology
Method used
Image
Examples
Embodiment
[0079] The present invention proposes a method for implementing a federated learning system oriented to the fusion of IoT and IoT in a smart city. The following is its code implementation part (interception is important):
[0080] As shown in Listing 1, this part includes the code implementation for the device to communicate with the server:
[0081]
[0082]
[0083]
[0084]
[0085]
[0086]
[0087]
[0088]
[0089]
[0090] Code 1 describes the process of communication between the server and the device. The server uses Flask to start the device registration service at startup and waits for the device to send a request. When the device is started, it sends a request to the server. After the server responds to the request, it registers the device information and assigns a number to the device, and subscribes to the corresponding MQTT topic according to the device number to establish a connection channel with the device. At the same time, the trai...
PUM
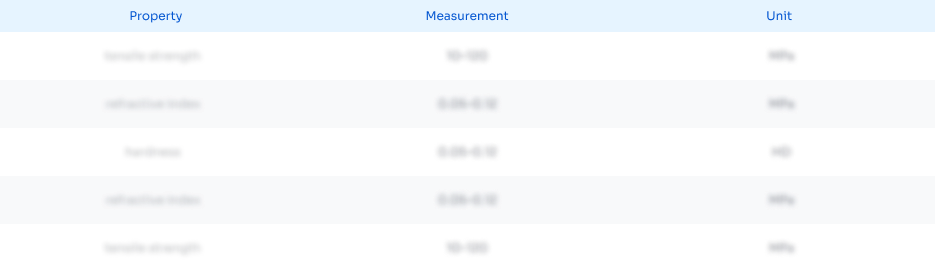
Abstract
Description
Claims
Application Information
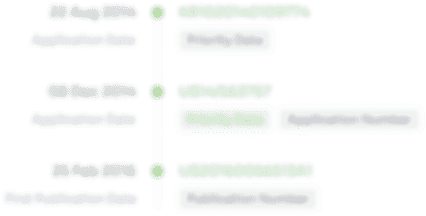
- R&D Engineer
- R&D Manager
- IP Professional
- Industry Leading Data Capabilities
- Powerful AI technology
- Patent DNA Extraction
Browse by: Latest US Patents, China's latest patents, Technical Efficacy Thesaurus, Application Domain, Technology Topic, Popular Technical Reports.
© 2024 PatSnap. All rights reserved.Legal|Privacy policy|Modern Slavery Act Transparency Statement|Sitemap|About US| Contact US: help@patsnap.com