Method and device for training scheduling model, and method and device for realizing collaborative driving
A scheduling model and collaborative driving technology, applied in the field of neural networks, can solve problems such as the inability to provide real-time and efficient road right allocation decision-making solutions, and the inability to deploy urban road network traffic systems efficiently and quickly, so as to shorten vehicle scheduling time and improve scheduling The effect of efficiency and quality
- Summary
- Abstract
- Description
- Claims
- Application Information
AI Technical Summary
Problems solved by technology
Method used
Image
Examples
Embodiment Construction
[0047] The steps shown in the flowcharts of the figures may be implemented in a computer system, such as a set of computer-executable instructions
[0051] The first vehicle in the embodiment of the present invention refers to a sample vehicle used for scheduling model training.
[0055] Step 105, determine the parameters of the scheduling model to be trained according to the calculated delay sum.
[0056] The embodiment of the present invention obtains a scheduling model that can be used for vehicle scheduling through offline sample data training.
[0059] The obtained high-dimensional state vector is processed through a preset first recurrent neural network.
[0063] Location, priority, speed, turn and route.
[0065]
[0069] When the total delay calculated by the scheduling model converges, keep the parameters of the scheduling model unchanged.
[0075] A computer program when executed by a processor implements the method of training a scheduling model as described above.
[00...
PUM
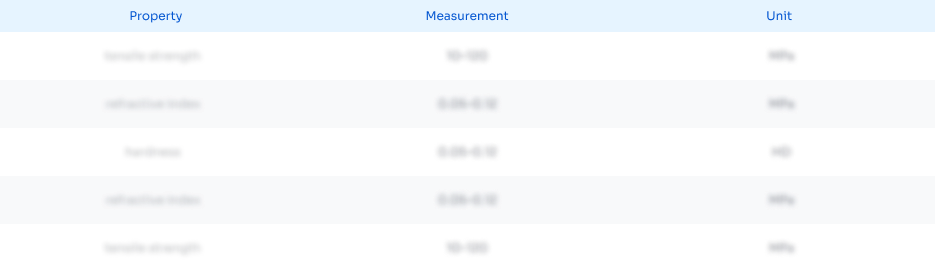
Abstract
Description
Claims
Application Information
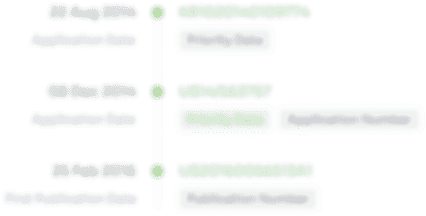
- R&D Engineer
- R&D Manager
- IP Professional
- Industry Leading Data Capabilities
- Powerful AI technology
- Patent DNA Extraction
Browse by: Latest US Patents, China's latest patents, Technical Efficacy Thesaurus, Application Domain, Technology Topic, Popular Technical Reports.
© 2024 PatSnap. All rights reserved.Legal|Privacy policy|Modern Slavery Act Transparency Statement|Sitemap|About US| Contact US: help@patsnap.com