Noise identification method for image data
A recognition method and image data technology, applied in the field of image recognition, can solve the problems of large loss value, low accuracy rate of noise samples, large difference between clean samples and category centers, etc., to achieve the effect of improving the accuracy rate
- Summary
- Abstract
- Description
- Claims
- Application Information
AI Technical Summary
Problems solved by technology
Method used
Image
Examples
Embodiment Construction
[0036] Embodiments of the present invention will be described in detail below. It should be emphasized that the following description is exemplary only, and is not intended to limit the scope of the invention and its application.
[0037] see figure 1 , an embodiment of the present invention provides a noise identification method for image data, including the following steps:
[0038] S1. In the noise learning based on neural network, a Gaussian mixture distribution model is used, and the combination of the loss value of the sample to be detected and the maximum non-target probability is used as a training dynamic to jointly model; wherein, the maximum non-target probability refers to, Among the classification and identification probabilities output by the sample after passing through the neural network, the probability is the largest except the target class probability. This largest non-target probability records the characteristics of the degree of confusion between the sam...
PUM
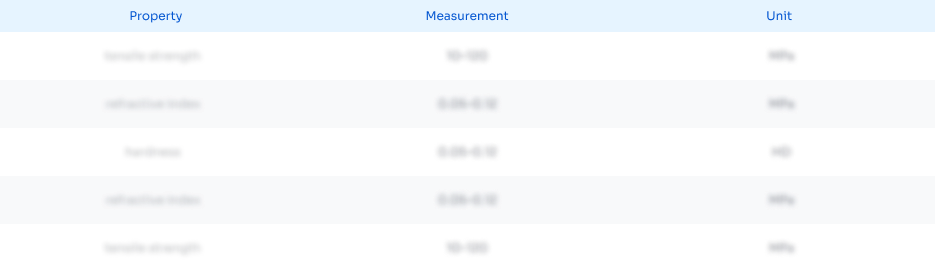
Abstract
Description
Claims
Application Information
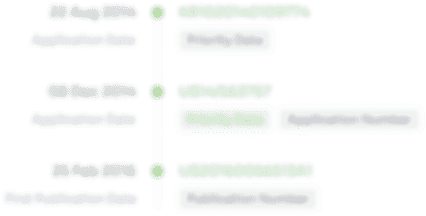
- R&D Engineer
- R&D Manager
- IP Professional
- Industry Leading Data Capabilities
- Powerful AI technology
- Patent DNA Extraction
Browse by: Latest US Patents, China's latest patents, Technical Efficacy Thesaurus, Application Domain, Technology Topic, Popular Technical Reports.
© 2024 PatSnap. All rights reserved.Legal|Privacy policy|Modern Slavery Act Transparency Statement|Sitemap|About US| Contact US: help@patsnap.com