Cell density classification method and device, electronic device and storage medium
A classification method and electronic device technology, applied in the field of computer learning, can solve the time-consuming problems of cell counting and achieve the effect of increasing speed
- Summary
- Abstract
- Description
- Claims
- Application Information
AI Technical Summary
Problems solved by technology
Method used
Image
Examples
Embodiment 3
[0070] Embodiment 3 Input the biological cell image to be tested into the training convolutional neural network model until the obtained reconstructed biological cell image matches the biological cell image to be tested, and determine that the cell density of the biological cell image to be tested is the same as the biological cell image to be tested. The density range corresponding to the training convolutional neural network model when reconstructing the biological cell image matching, therefore, in this case, the training convolutional neural network model is used to determine the cell density of the biological cell image to be tested, and the number of cells can be determined without calculating the number of cells. The cell density range is increased, and the speed of the cell count is increased.
[0071] Image 6 This is a flow chart of the cell density classification method provided in the fourth embodiment of the present invention. The cell density classification meth...
Embodiment 4
[0083] Embodiment 4 Acquire multiple training biological cell images divided into multiple different density ranges, and input multiple training biological cell images of each density range into different convolutional neural network models to obtain multiple training convolutional neural network models , input the biological cell image to be tested into the training convolutional neural network model until the obtained reconstructed biological cell image matches the biological cell image to be tested, and determine that the cell density of the biological cell image to be tested is the same as the reconstructed biological cell image. The density range corresponding to the training convolutional neural network model when matching biological cell images. Therefore, in this case, by first training the convolutional neural network model, and then determining the cell density of the biological cell image to be tested according to the training convolutional neural network model, the ...
PUM
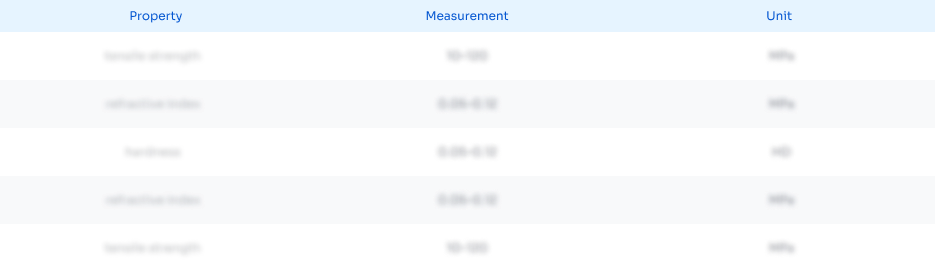
Abstract
Description
Claims
Application Information
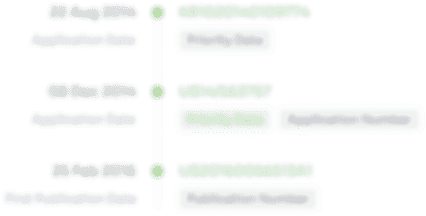
- R&D Engineer
- R&D Manager
- IP Professional
- Industry Leading Data Capabilities
- Powerful AI technology
- Patent DNA Extraction
Browse by: Latest US Patents, China's latest patents, Technical Efficacy Thesaurus, Application Domain, Technology Topic, Popular Technical Reports.
© 2024 PatSnap. All rights reserved.Legal|Privacy policy|Modern Slavery Act Transparency Statement|Sitemap|About US| Contact US: help@patsnap.com