Data enhancement method for small-scale target
A target enhancement, small-scale technology, applied in the field of deep learning, can solve the problems of small proportion of data sets, unbalanced samples, and few available features, so as to alleviate the unbalanced samples, improve the overall performance, and enhance the robustness.
- Summary
- Abstract
- Description
- Claims
- Application Information
AI Technical Summary
Problems solved by technology
Method used
Image
Examples
Embodiment Construction
[0033] The present invention will be further described in detail below in conjunction with the accompanying drawings and specific embodiments.
[0034] see figure 1 , a data enhancement method for small-scale targets according to an embodiment of the present invention, comprising the following steps:
[0035] S1: Collect and read small-scale targets;
[0036] S11: Determine the small-scale target according to the definition of the small scale, that is, the ratio of the pixels of each target to the total pixels in the data set, and collect the small-scale targets;
[0037] S12: Since there may be multiple small-scale targets in an image, (see figure 2 a), so select a suitable small-scale target as the object of replication according to the requirements; convert the collected small-scale target into a standard image format;
[0038] S13: Scale the collected small-scale targets to increase the robustness of small-scale targets; at the same time, use triple linear interpolatio...
PUM
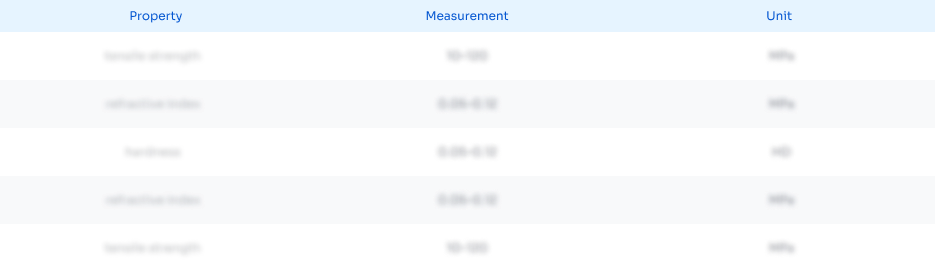
Abstract
Description
Claims
Application Information
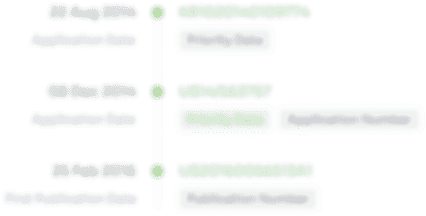
- R&D Engineer
- R&D Manager
- IP Professional
- Industry Leading Data Capabilities
- Powerful AI technology
- Patent DNA Extraction
Browse by: Latest US Patents, China's latest patents, Technical Efficacy Thesaurus, Application Domain, Technology Topic.
© 2024 PatSnap. All rights reserved.Legal|Privacy policy|Modern Slavery Act Transparency Statement|Sitemap