Small sample rolling bearing fault diagnosis method under multiple working conditions
A rolling bearing and fault diagnosis technology, which is applied in the field of diagnosis, can solve the problems of increasing the difficulty of intelligent diagnosis of bearing faults, complex fault conditions, and difficulty in improving recognition accuracy, etc., to improve sparse representation capabilities, improve anti-noise capabilities, and improve generalization effect of ability
- Summary
- Abstract
- Description
- Claims
- Application Information
AI Technical Summary
Problems solved by technology
Method used
Image
Examples
Embodiment Construction
[0032] The invention provides a small-sample rolling bearing fault diagnosis method under multiple working conditions combined with MAML and ISDAE. The method can effectively extract deep element features of rolling bearing faults under multiple working conditions, and then realize accurate identification of rolling bearing fault states.
[0033] Such as figure 1 Shown, the present invention comprises the following steps:
[0034] 1) First, in order to meet the needs of meta-learning tasks, the original vibration signals are classified according to different working conditions, different sampling frequencies, different fault degrees, and different fault types to construct a task set.
[0035] 2) Input the task set data constructed in step 1) into ISDAE for reconstruction, given the data sample matrix composed of original vibration signals where x m Represents the mth sample, M represents the number of samples, (i=1,2,...n) represents the i-th data of the m-th sample, and ...
PUM
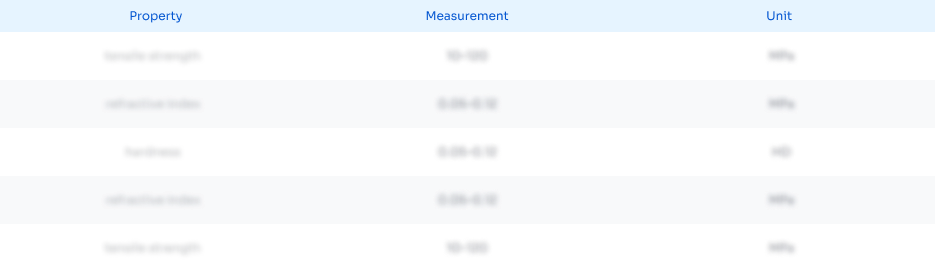
Abstract
Description
Claims
Application Information
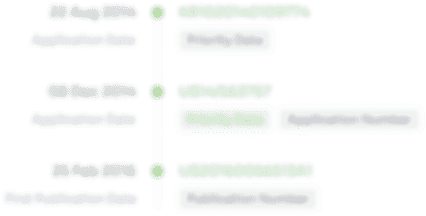
- R&D Engineer
- R&D Manager
- IP Professional
- Industry Leading Data Capabilities
- Powerful AI technology
- Patent DNA Extraction
Browse by: Latest US Patents, China's latest patents, Technical Efficacy Thesaurus, Application Domain, Technology Topic, Popular Technical Reports.
© 2024 PatSnap. All rights reserved.Legal|Privacy policy|Modern Slavery Act Transparency Statement|Sitemap|About US| Contact US: help@patsnap.com