Emotion recognition method based on self-training maximization classifier difference
An emotion recognition and classifier technology, applied in the field of emotion recognition, can solve the problems of declining classification effect and ignoring spatial information, and achieve the effect of accurate emotion recognition results and good economic benefits.
- Summary
- Abstract
- Description
- Claims
- Application Information
AI Technical Summary
Problems solved by technology
Method used
Image
Examples
specific Embodiment approach
[0059] A specific implementation of the training of the emotion recognition model based on the self-training maximization classifier difference is as follows:
[0060] The process from the original EEG data set to the final construction of 3D cube features is as follows: figure 2 As shown, the specific process is:
[0061] Preprocessing of raw EEG data: downsampling of raw EEG data on SEED and SEED-IV datasets from 1000 Hz to 200 Hz; further filtering out noise and removing artifacts using bandpass filtering at 0.3-75 Hz. The non-overlapping EEG signals filtered by each channel on the preprocessed SEED and SEED-IV datasets are divided into 1s and 4s segments respectively; these segments have their corresponding emotional labels.
[0062] The obtained fragments are filtered in five frequency bands; the five frequency bands are: delta (1-3Hz), theta (4-7Hz), alpha (8-13Hz), beta (14-30Hz) and gamma (31-50Hz ); Extract differential entropy (DE) features to five frequency bands...
PUM
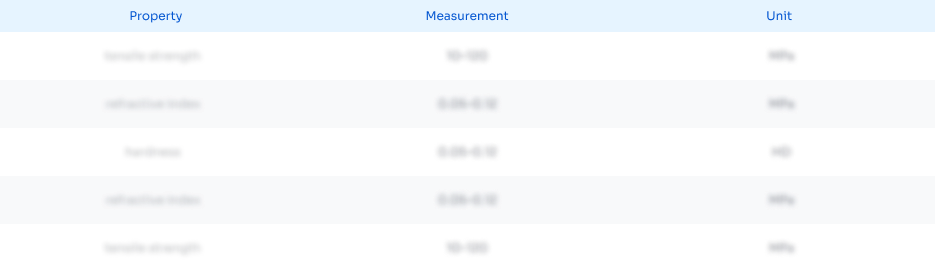
Abstract
Description
Claims
Application Information
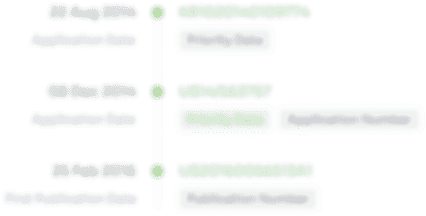
- R&D Engineer
- R&D Manager
- IP Professional
- Industry Leading Data Capabilities
- Powerful AI technology
- Patent DNA Extraction
Browse by: Latest US Patents, China's latest patents, Technical Efficacy Thesaurus, Application Domain, Technology Topic.
© 2024 PatSnap. All rights reserved.Legal|Privacy policy|Modern Slavery Act Transparency Statement|Sitemap